X射线吸收光谱被广泛应用于探测吸收原子周围的局部原子和电子结构。X射线近边吸收结构包含结构描述符的多种信息,如键长、键角、配体类型、氧化态等,它们将会影响光谱描述符,包括边缘位置、光谱最大值和最小值的形状、位置等。然而,对于光谱的定量分析并不简单。目前,人们在光谱定量分析方面取得了一些进展。但对于特定光谱来说,改进哪些结构参数以及如何估计不确定性还面临着困难。
来自俄罗斯南方联邦大学智能材料研究所的Guda等,提出了一种用于分析X射线近边吸收结构光谱的替代概念。以机器学习算法为基础,利用描述符直观表征光谱的形状,包括边缘的能量位置、最大值和最小值的强度和曲率等。通过机器学习,作者分析了确定描述符的合理组合,并且结合散点图推导出了光谱描述符和结构参数之间的分析依赖关系,这使光谱-结构关系得以可视化。这种方法克服了理论光谱和实验光谱之间存在的系统差异。此外,数值关系可以用解析公式表达,为基于光谱形状的结构参数提取提供了一种简单快速的工具。该方法被成功地应用于多组分Fe: SiO2系统和含铁的化合物参照样品中,证明了理论验证集和实验数据的高质量预测。该文近期发表于
npj Computational Materials 7, 203 (2022),英文标题与摘要如下,点击左下角“阅读原文”可以自由获取论文PDF。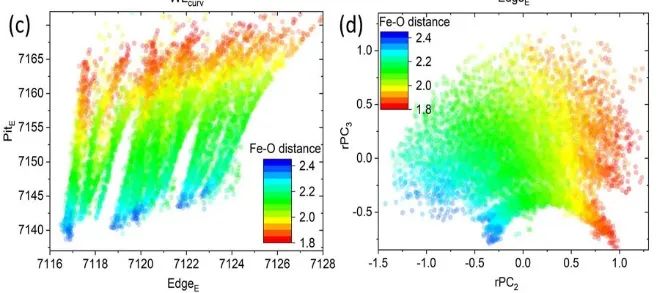
Understanding X-ray absorption spectra by means of descriptors and machine learning algorithms
A. A. Guda, S. A. Guda, A. Martini, A. N. Kravtsova, A. Algasov, A. Bugaev, S. P. Kubrin, L. V. Guda, P. Šot, J. A. van Bokhoven, C. Copéret & A. V. Soldatov
X-ray absorption near-edge structure (XANES) spectra are the fingerprint of the local atomic and electronic structures around the absorbing atom. However, the quantitative analysis of these spectra is not straightforward. Even with the most recent advances in this area, for a given spectrum, it is not clear a priori which structural parameters can be refined and how uncertainties should be estimated. Here, we present an alternative concept for the analysis of XANES spectra, which is based on machine learning algorithms and establishes the relationship between intuitive descriptors of spectra, such as edge position, intensities, positions, and curvatures of minima and maxima on the one hand, and those related to the local atomic and electronic structure which are the coordination numbers, bond distances and angles and oxidation state on the other hand. This approach overcomes the problem of the systematic difference between theoretical and experimental spectra. Furthermore, the numerical relations can be expressed in analytical formulas providing a simple and fast tool to extract structural parameters based on the spectral shape. The methodology was successfully applied to experimental data for the multicomponent Fe: SiO2 system and reference iron compounds, demonstrating the high prediction quality for both the theoretical validation sets and experimental data.
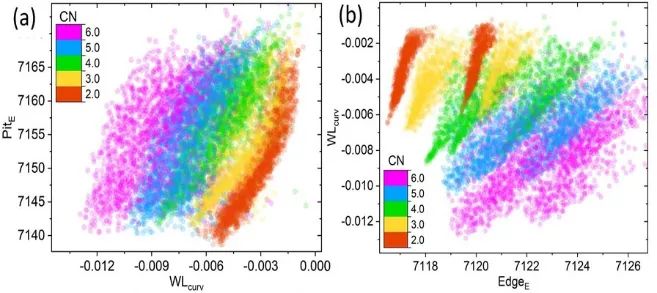