香港浸会大学物理系理论与计算研究团队由汤雷翰教授、周昌松教授、唐乾元博士、田亮博士和曾祥泽博士组成。团队的主要科研方向是开发和应用先进的统计力学和计算的方法来研究生物系统中的复杂动力学现象,尺度跨越从蛋白质到人类大脑。 与行为和疾病研究密切相关的大规模数据分析和建模是我们团队研究中的关键部分。目前团队正在招收统计物理,机器学习,计算生物学,脑科学方向博士生,欢迎感兴趣的同学加入!
The Theoretical and Computational Research Cluster in the Physics Department, Hong Kong Baptist University consists of Drs. Leihan Tang, Qianyuan Tang, Liang Tian, Xiangze Zeng, and Changsong Zhou. Main focus of the group is the development and application of advanced statistical mechanical and computational methods to study complex dynamical phenomena in biological systems, ranging from proteins to the human brain. Large-scale data analysis and modelling, in close association with behavioral and disease studies, is a key part of the cluster’s research agenda.
1. Bioinformatics, Machine Learning, and Network Science (Liang Tian, Qianyuan Tang, Leihan Tang)
2. Complex Neural Dynamics and Computation (Changsong Zhou, Liang Tian, Qianyuan Tang)
3. Human Microbiome and Community Ecology (Liang Tian)
4. Intrinsically Disordered Proteins and Phase Separation in Cells (Xiangze Zeng)
5. Protein Dynamics and Evolution (Qianyuan Tang)
6. Statistical Physics of Networks: Model Construction and Analysis (Leihan Tang)
7. TCM Pharmacological principles and their applications in human diseases and drug-disease relationship (Liang Tian, Leihan Tang)
[1] Bioinformatics, Machine Learning, and Network Science (Liang Tian, Qianyuan Tang, Leihan Tang)
The exponential growth of the amount of data available today prompts us to adopt and develop techniques and algorithms to transform all these heterogeneous data into universal knowledge and testable models. We are generally interested in multi-dimensional multi-omics biological data analysis modeling using various techniques from machine learning, deep learning, network science, etc.L. Tian, et al., Articulation points in complex networks, Nature Communications, 8, 14223 (2017).
- L. Tian, X.-F. Li, F Qi, Q.-Y. Tang, V. Tang, J. Liu, Z.-Y. Li, X.-Y. Cheng, X.-X. Li, Y.-C. Shi, H.-G. Liu, L.-H. Tang, Harnessing peak transmission around symptom onset for non-pharmaceutical intervention and containment of the COVID-19 pandemic, Nature Communications, 12, 1147 (2021).
[2] Complex Neural Dynamics and Computation (Changsong Zhou, Liang Tian, Qianyuan Tang)Neural network connectivity and activity in the brain to support advanced brain functioning are highly complex. With advanced technology, cognitive and neurosciences have moved into the big data era, and deciphering neural complexity has become a grand challenge in brain science. We aim to study the structure-dynamics-function relationships of neural systems using approaches of statistical physics and complex dynamical network systems, by integrating computational modeling and analysis of various experimental data, such as EEG, fMRI, PET. Our focus is on neural complexity, including the organization principles (e.g., cost efficiency in structure and activity) and dynamical mechanism (e.g., neural criticality) of spontaneous and evoked complex neural activity at different scales (local circuit vs. whole brain connectome) and their impacts in neural information processing (e.g., learning and memory), brain cognition (e.g., cognitive flexibility) and neuropsychiatric disorders. We are also interested in applying the understanding from biological neural networks to the development of human-like, cost-efficient AI rooted in neural complexity. Our projects include: 1) Cost-efficient neural dynamics, intricate interaction with neural plasticity and information processing in neural circuit models; 2) Analyzing and modeling large-scale brain structural and functional networks in health and disorders, and applications in network-based brain modulations; and 3) Machine learning with complex neural network structure and dynamics. These projects will be carried out in close collaboration with scientists from computational and experimental cognitive and neurosciences, and experts in machine learning and AI.J.H. Liang and C.S. Zhou, Criticality enhances the multilevel reliability of stimulus responses in cortical neural networks, PLoS Compuational Biology, 18, e1009848 (2022).
J.H. Liang, S.J. Wang, and C.S. Zhou. Less is more: wiring-economical modular networks support self-sustained firing-economical neural avalanches for efficient processing, National Science Review, 9, nwab102 (2022).
R. Wang, M.X. Liu, X. Cheng, Y. Wu, A. Hildebrandt, and C.S. Zhou. Segregation, integration and balance of large-scale resting brain networks configure different cognitive abilities, Proc Natl Acad Sci USA, 118, e2022288118 (2021).
Z.D. Bi and C.S. Zhou, Understanding the computation of time using neural network models, Proc Natl Acad Sci USA, 117, 10530 (2020).
- R. Wang, P. Lin, M.X. Liu, Y. Wu, T Zhou and C.S. Zhou, Hierarchical connectome modes and critical state jointly maximize human brain functional diversity. Physical Review Letters 123, 038301
[3] Human Microbiome and Community Ecology (Liang Tian)A variety of microbial communities exist throughout the human body, which play a crucial role in determining our health and well-being. The alterability of our microbiome offers a promising future for a variety of microbiome-based therapies such as ingesting probiotics or prebiotics, and fecal microbiota transplantation, in treating diseases associated with disrupted microbiota. However, since the microbiome is an extremely complex and dynamic ecological system, we still lack sufficient understanding of its organizational and ecological features and principles in order to rationally and precisely design its manipulations. In this project, we aim to characterize the complex topology and dynamics of the ecological network underlying the human gut microbiota and construct modeling frameworks to better capture its community ecology toward developing effective, stable, and personalized microbiome-based therapies.L. Tian, et al., Deciphering Functional Redundancy in the Human Microbiome, Nature Communications, 11, 6217 (2020)
- M. Chen, Z. Wei, L. Tian, Y. Tan, J.-D. Huang, L. Dai, Design and application of synthetic microbial communities, Chinese Science Bulletin, 1950015 (2020) (Review paper)
[4] Intrinsically Disordered Proteins and Phase Separation in Cells (Xiangze Zeng)Macromolecular phase separation has become a new principle to understand cellular functions. It underlies the formation of membraneless organelles and regulates many cellular processes. Dysregulation of phase separation in cells is implicated with various diseases including cancers and neurodegenerative diseases. It has been found that many intrinsically disordered proteins (IDPs) can undergo phase separation coupled to percolation (PSCP) and form biomolecular condensates. We are interested in understanding the physics of single-chain behavior of IDPs, the multivalent interactions mediated by the intrinsically disordered domains, and phase separation processes involving IDPs, RNA and DNA molecules. Molecular dynamics and Monte Carlo simulations, and theories of phase transitions and polymer physics will be used. This study will provide new insights into the treatment of diseases involving phase separation processes.X. Zeng, K. M. Ruff, R. V. Pappu, "Competing interactions give rise to two-state behavior and switch-like transitions in charge-rich intrinsically disordered proteins", Proc. Natl. Acad. Sci. U.S.A., 119(19)e2200559119 (2022)
X. Zeng, C. Liu, M. J. Fossat, P. Ren, A. Chilkoti, R. V. Pappu, "Design of intrinsically disordered proteins that undergo phase transitions with lower critical solution temperatures", APL Material, 9, 021119 (2021)
- X. Zeng, A. S. Holehouse, A. Chiltoki, T. Mittag, R. V. Pappu, "Connecting Coil-to-Globule Transitions to Full Phase Diagrams for Intrinsically Disordered Proteins", Biophys. J., 119 (2), 402-418 (2020)
[5] Protein Dynamics and Evolution (Qianyuan Tang)The genotype-phenotype mapping of proteins is a fundamental question in structural biology. Proteins possess flexible structures that allow them to adapt to the environments and perform their dynamics related to biological functions. Meanwhile, the mutations in protein sequences may lead to variations in the protein structures, resulting in changes in their dynamics. The recent development of artificial intelligence (AI) provides us with new and powerful tools to analyze protein dynamics, evolution, and their correspondence on a large scale. The analyses based on AI-predicted protein structures, integrated with other kinds of biological big data, will not only have the potential to answer critical questions in the survival and regulation mechanisms of cells, but also offer new insights into evolutionary biology and protein design.Q.-Y. Tang, W. Ren, J. Wang, K. Kaneko, The Statistical Trends of Protein Evolution: A Lesson from AlphaFold Database, BioRxiv 10.1101/2022.04.07.487447
(2022).
Q.-Y. Tang, K. Kaneko. Dynamics-Evolution Correspondence of Protein Structures. Physical Review Letters, 127, 098103 (2021).
Q.-Y. Tang, T. S. Hatakeyama, K. Kaneko, Functional Sensitivity and Mutational Robustness of Proteins, Physical Review Research, 2(3), 033452 (2020).
- Q.-Y. Tang, K. Kaneko. Long-range correlation in protein dynamics: Confirmation by structural data and normal mode analysis. PLoS Computational Biology, 16(2), e1007670 (2020).
[6] Statistical Physics of Networks: Model Construction and Analysis (Leihan Tang)Networks play an essential role in the quantitative description of collective phenomena in natural and artificial systems. The dynamical behavior of such systems is extremely rich, ranging from synchronized motion to large-scale patterns that evolve over time. In biological and social systems, individual nodes in the network are themselves multimodal entities able to engage in different tasks in response to environmental cues. Recent projects in the group include pattern formation in engineered bacterial colonies, collective oscillations in adaptive cell populations and active bacterial thin films, embryogenesis of C. elegans, and COVID-19 pandemic waves in urban settings. We are also interested in application and development of modern statistical physics techniques and methodologies for data processing and network reconstruction, such as time-series data analysis and spectral methods. Progress in this direction will improve corresponding statistical physics models, extract universal properties and physical mechanisms, and explore new ideas for discussing the relationship between network structure and function.L. Tian, X.-F. Li, F Qi, Q.-Y. Tang, V. Tang, J. Liu, Z.-Y. Li, X.-Y. Cheng, X.-X. Li, Y.-C. Shi, H.-G. Liu, L.-H. Tang, Harnessing peak transmission around symptom onset for non-pharmaceutical intervention and containment of the COVID-19 pandemic, Nat Comm, 12, 1147 (2021).
G. Guan, M.-K. Wong, Z. Zhao, L.-H. Tang, and C. Tang, “Volume segregation programming in a nematode's early embryogenesis,” Phys. Rev. E 104, 054409 (2021).
S.-W. Wang and L.-H. Tang, “Emergence of collective oscillations in adaptive cells”, Nat Comm, 10, 5613 (2019).
S.-W. Wang, K. Kawaguchi, S.-i. Sasa, and L.-H. Tang, “Entropy production of nanosystems with time scale separation”, Phys Rev Lett, 117, 070601 (2016).
[7] TCM Pharmacological principles and their applications in human diseases and drug-disease relationship (Liang Tian, Leihan Tang)Traditional Chinese Medicine (TCM) characterizes and addresses human physiology, pathology, and diseases diagnosis and prevention using TCM theories and Chinese herbal products. However, the pharmacological principles in TCM theory, the core treasure house of TCM, have rarely been systematically investigated in a top-down manner, which hinders the modernization and standardization of TCM. In this project, we will establish a novel TCM-based network pharmacology framework to discern low-dimensional patterns and principles of human disease and herb-diseases associations. This project will provide a basis for the objectification of the diagnosis and treatment process of TCM, and the knowledge fusion of TCM evidence-based medicine and modern biology.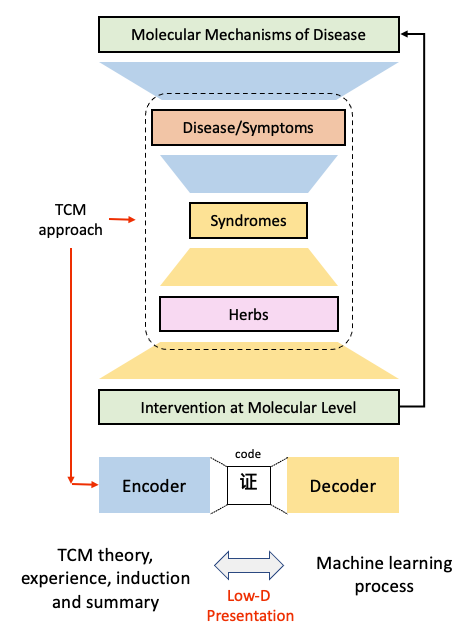
The ideal candidate is expected to have a background in one or more of the following fields: physics, biology, chemistry, computer science, or mathematics.Excellent organizational and interpersonal skills, along with a stated interest in scientific research, are essential. Computer programming and analytic experience with large datasets is a plus.
All interested candidates should submit an application consisting of (i) current Curriculum Vitae, (ii) brief statement of research experience and interests, and (iii) transcripts (as one single PDF file) to