海归学者发起的公益学术平台
分享信息,整合资源
交流学术,偶尔风月
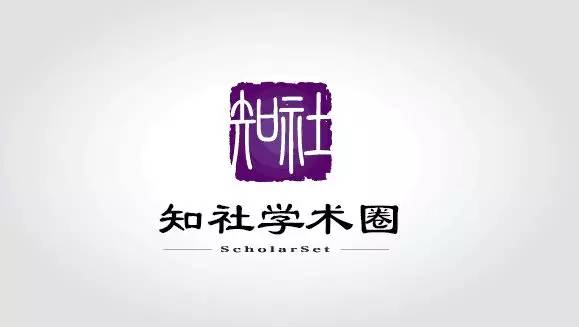
单线态裂变(SF)是指一个受光激发产生的单线态激子转变为两个三线态激子的过程。SF材料可用于太阳能电池中,利用高能光子的过剩能量并减少热耗散引起的能量损失,从而显著提高太阳能电池的光电转换效率。目前,能够发生单线态裂变的分子晶体非常稀少。理论计算可以加速这类材料的发现,但由于高额的计算成本,用于计算分子晶体中激子特性的多体微扰理论(MBPT)对于大规模的材料筛选来说并不实用。一种可行的思路是采用第一性原理模拟体系的主要物理化学特征,并利用机器学习算法进行特征筛选,从而建立能够快速评估并准确预测MBPT结果的计算模型。
来自卡内基梅隆大学材料科学与工程系的Noa Marom教授研究团队,使用确定独立筛选和稀疏算子(SISSO)机器学习算法,建立了预测多体微扰理论中SF热力学驱动力的高效计算模型,并将该应用于101种多环芳烃构成的数据集中。SISSO算法通过不断组合主要的物理特征进行建模,并通过线性回归以及交叉验证筛选出最优模型。SISSO模型成功地预测能发生单线态裂变的驱动力,误差范围低于0.2 eV。基于SISSO模型的成本、准确性和分类性能,该研究团队提出了一种分层材料筛选流程,并在PAH101数据集中找到了三个潜在的SF候选材料。这些候选材料迄今为止还尚未在SF材料背景下被研究过,将有助于引导相关的实验工作。该文近期发表于npj Computational Materials 8:70(2022),英文标题与摘要如下,点击左下角“阅读原文”可以自由获取论文PDF。
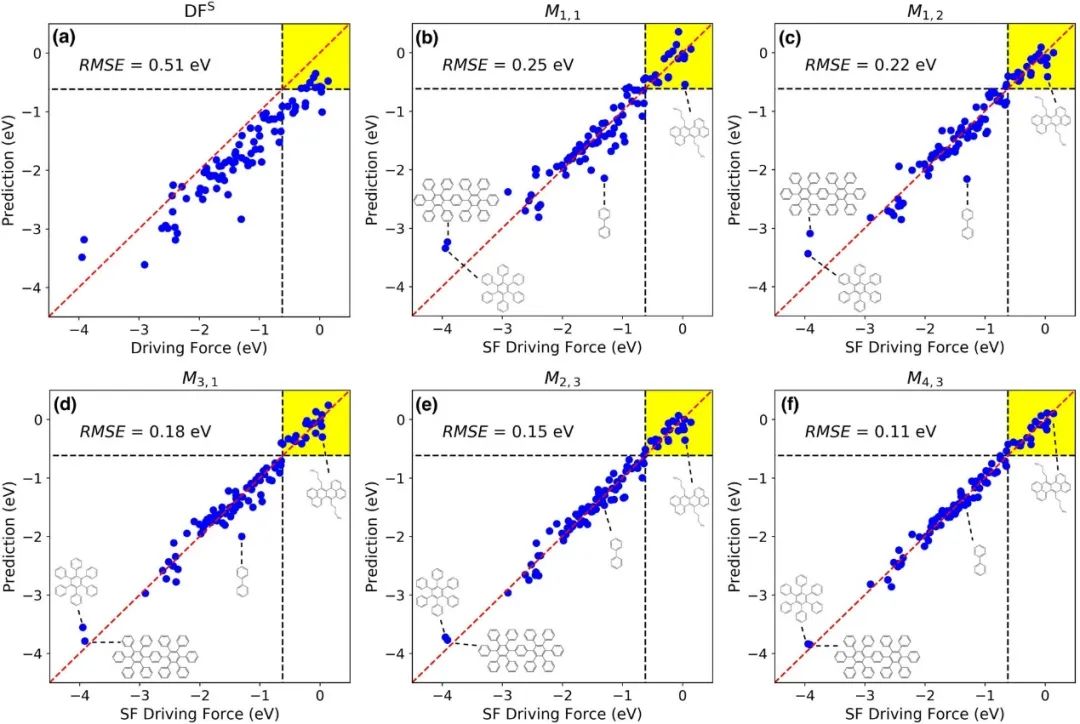
Finding predictive models for singlet fission by machine learning
Xingyu Liu, Xiaopeng Wang, Siyu Gao, Vincent Chang, Rithwik Tom, Maituo Yu, Luca M. Ghiringhelli & Noa Marom
Singlet fission (SF), the conversion of one singlet exciton into two triplet excitons, could significantly enhance solar cell efficiency. Molecular crystals that undergo SF are scarce. Computational exploration may accelerate the discovery of SF materials. However, many-body perturbation theory (MBPT) calculations of the excitonic properties of molecular crystals are impractical for large-scale materials screening. We use the sure-independence-screening-and-sparsifying-operator (SISSO) machine-learning algorithm to generate computationally efficient models that can predict the MBPT thermodynamic driving force for SF for a dataset of 101 polycyclic aromatic hydrocarbons (PAH101). SISSO generates models by iteratively combining physical primary features. The best models are selected by linear regression with cross-validation. The SISSO models successfully predict the SF driving force with errors below 0.2 eV. Based on the cost, accuracy, and classification performance of SISSO models, we propose a hierarchical materials screening workflow. Three potential SF candidates are found in the PAH101 set.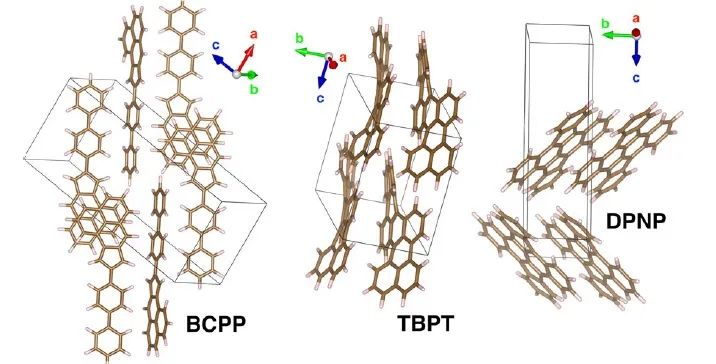