Can we use machine learning methods to predict the sensing data of odor mixtures and design new smells? A new study by researchers from Tokyo Tech does just that. The novel method is bound to have applications in the food, health, beauty, and wellness industries, where odors and fragrances are of keen interest.
我们能否使用机器学习方法来预测气味混合物的传感数据并设计新的气味?东京工业大学研究人员的一项新研究就是这样做的。这种新方法必将应用于食品、健康、美容和保健行业,在这些行业中,气味和香料引起了人们的极大兴趣。
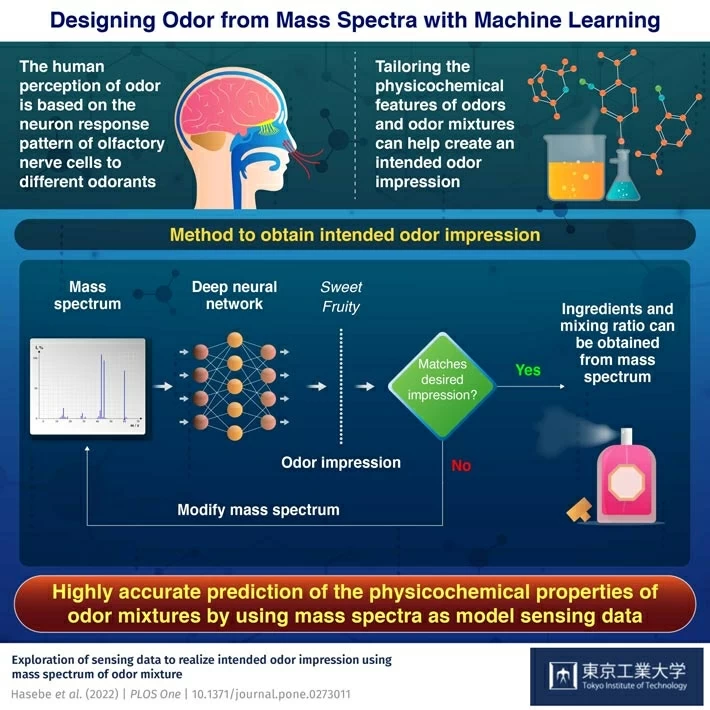
The sense of smell is one of the basic senses of animal species. It is critical to finding food, realizing attraction, and sensing danger. Humans detect smells, or odorants, with olfactory receptors expressed in olfactory nerve cells. These olfactory impressions of odorants on nerve cells are associated with their molecular features and physicochemical properties. This makes it possible to tailor odors to create an intended odor impression. Current methods only predict olfactory impressions from the physicochemical features of odorants. But, that method cannot predict the sensing data, which is indispensable for creating smells.
嗅觉是动物物种的基本感觉之一。它对寻找食物、意识到吸引力和感知危险至关重要。人类通过在嗅觉神经细胞中表达的嗅觉感受器来探测气味或气味。这些气味对神经细胞的嗅觉印象与其分子特征和物理化学性质有关。这使得定制气味成为可能,以创造一种预期的气味印象。目前的方法只能从气味的物理化学特征来预测嗅觉印象。但是,这种方法无法预测气味产生所必需的传感数据。
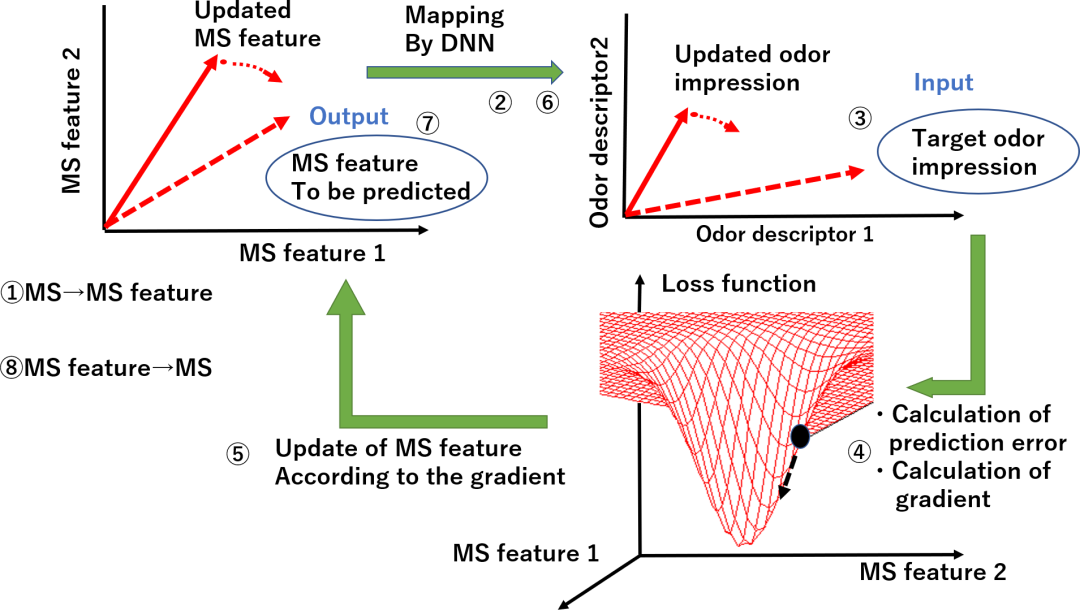
从气味印象到质谱的预测原理
To tackle this issue, scientists from Tokyo Institute of Technology (Tokyo Tech) have employed the innovative strategy of solving the inverse problem. Instead of predicting the smell from molecular data, this method predicts molecular features based on the odor impression. This is achieved using standard mass spectrum data and machine learning (ML) models. "We used a machine-learning-based odor predictive model that we had previously developed to obtain the odor impression. Then we predicted the mass spectrum from odor impression inversely based on the previously developed forward model," explains Professor Takamichi Nakamoto, the leader of the research effort by Tokyo Tech. The findings have been published in PLoS One.
为了解决这个问题,东京工业大学(Tokyo Tech)的科学家们采用了解决逆问题的创新策略。该方法不是根据分子数据预测气味,而是根据气味印象预测分子特征。这是使用标准质谱数据和机器学习(ML)模型实现的。“我们使用了之前开发的基于机器学习的气味预测模型来获得气味印象。然后,我们根据之前开发的正向模型,从气味印象中反向预测了质谱,”东京工业大学研究项目的负责人中本孝道教授解释道。研究结果已发表在PLoS One杂志上。
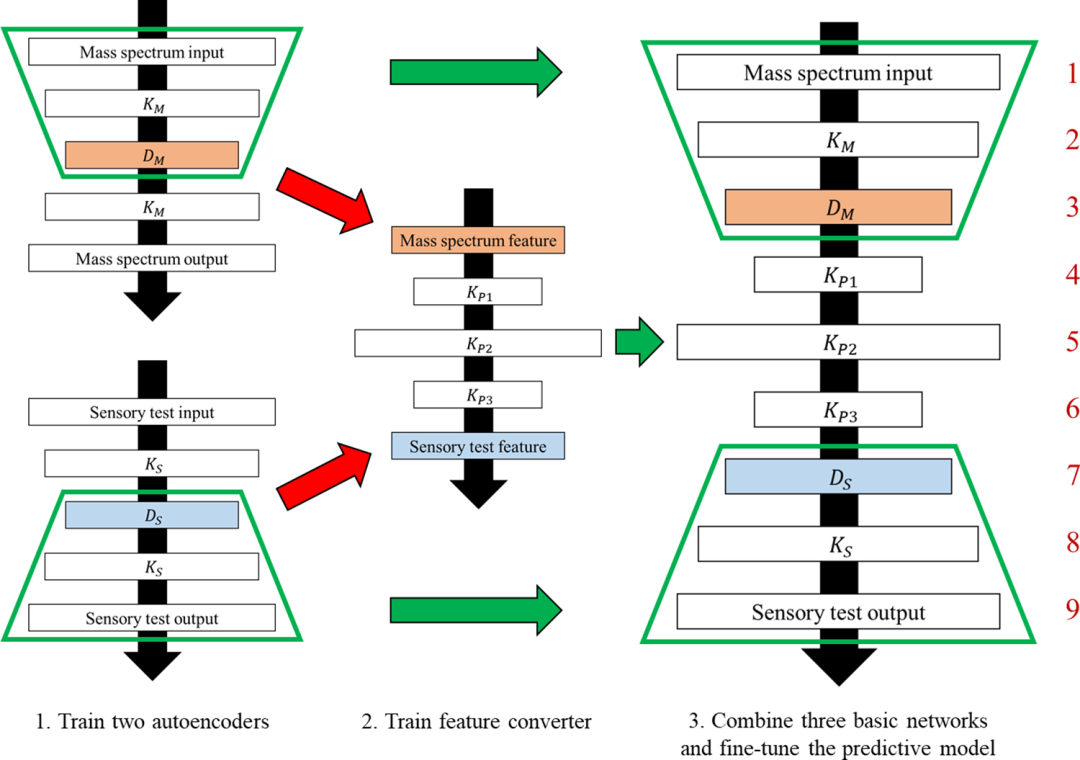
气味印象预测模型示意图
The mass spectra of odor mixtures is obtained by a linear combination of the mass spectra of single components. This simple method allows for the quick preparation of the predicted spectra of odor mixtures and can also predict the required mixing ratio, an important part of the recipe for new odor preparation. "For example, we show which molecules give the mass spectrum of apple flavor with enhanced 'fruit' and 'sweet' impressions. Our analysis method shows that combinations of either 59 or 60 molecules give the same mass spectrum as the one obtained from the specified odor impression. With this information, and the correct mixing ratio needed for a certain impression, we could theoretically prepare the desired scent," highlights Prof. Nakamoto.
气味混合物的质谱是由单一组分的质谱线性组合得到的。这种简单的方法可以快速制备气味混合物的预测色谱,也可以预测所需的混合比例,这是新气味制备配方的重要组成部分。“例如,我们展示了哪些分子使苹果味的质谱增强了‘水果’和‘甜’的印象。我们的分析方法表明,59或60个分子的组合与从指定的气味印象中获得的质谱相同。有了这些信息,以及特定印象所需的正确混合比例,理论上我们可以制备出所需的气味,”中本教授强调说。
This novel method described in this study can provide highly accurate predictions of the physicochemical properties of odor mixtures, as well as the mixing ratios required to prepare them, thereby opening the door to endless tailor-made fragrances.
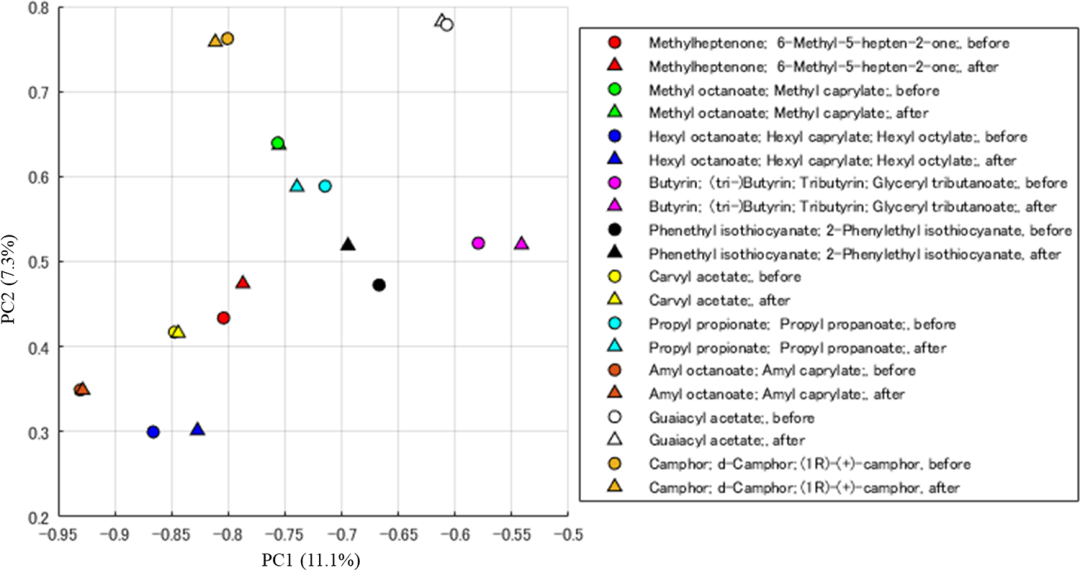
对DREAM数据集中的10个分子应用梯度下降算法前后MS特征的PCA图。圆形是将原质谱降维后得到的质谱特征。三角形是用梯度下降法搜索的质谱特征,由气味印象预测。所有的图都是用第一主成分和第二主成分和方差比例得到的。
本研究中描述的这种新方法可以非常准确地预测气味混合物的物理化学性质,以及制备它们所需的混合比例,从而为无尽的定制香料打开了大门。
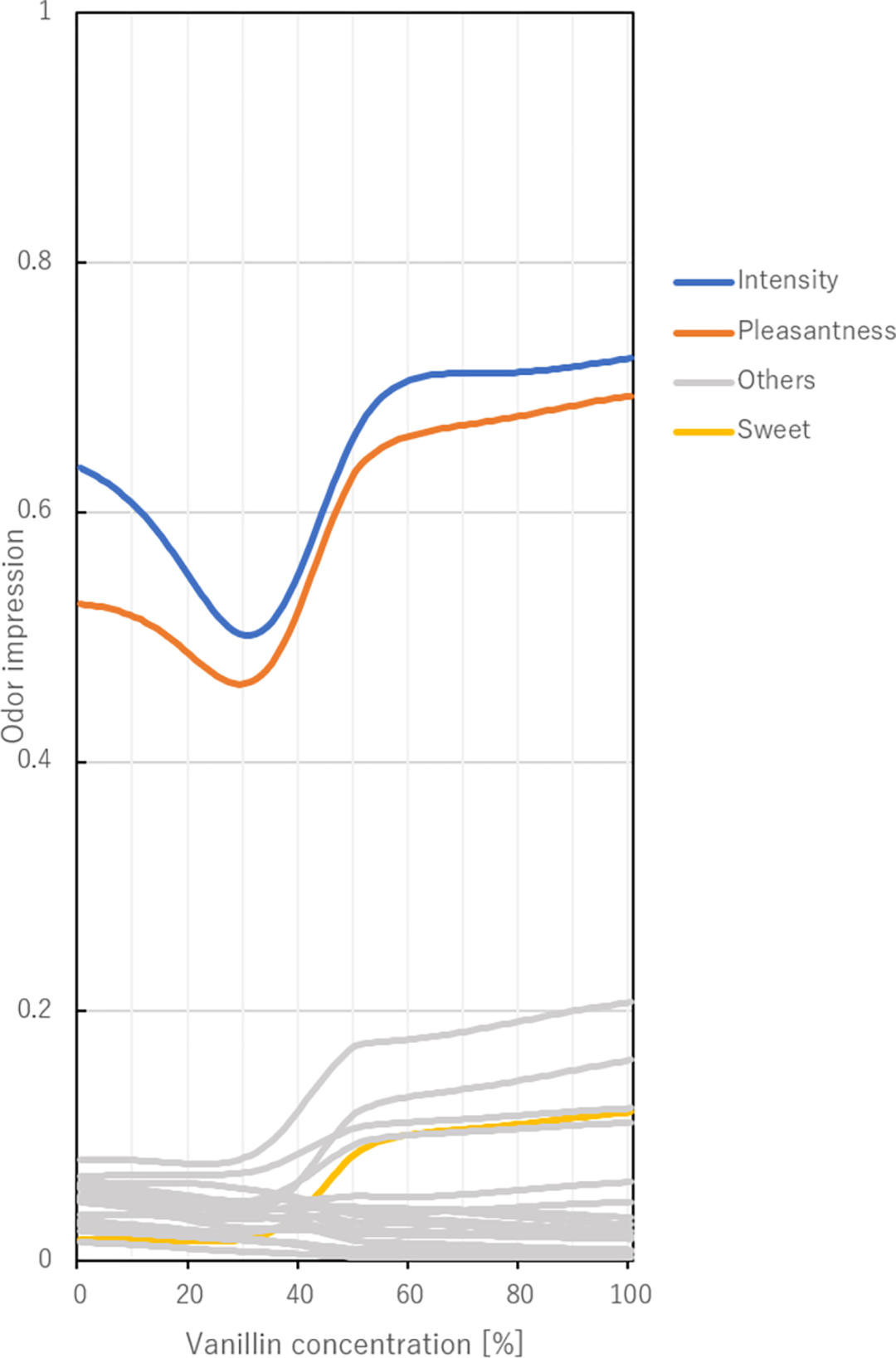
每种香草醛和柠檬醛混合比例的气味描述符分数的变化。横轴为香兰素的混合比例。
It looks like the future of odor mixtures smells good!
看起来未来的气味混合物很好闻!
Reference
Authors : | Daisuke Hasebe1, Manuel Alexandre1,2, and Takamichi Nakamoto1 |
Title of original paper : |
Exploration of sensing data to realize intended odor impression using mass spectrum of odor mixture |
Journal : | PLOS One |
DOI : | 10.1371/journal.pone.0273011 |
Affiliations : | 1School of Engineering, Tokyo Institute of Technology, Yokohama, Kanagawa, Japan 2Institute of Innovation Research, Tokyo Institute of Technology, Kanagawa, Japan |
* Corresponding author's email: nakamoto@mn.ee.titech.ac.jp