小编的话:本文的作者龚鹤扬是中科大统计学的博士,他之前曾建议我翻译下Hernan(2019) A Second Chance to Get Causal Inference Right: A Classification of Data Science Tasks,这篇文,我很欣赏这篇文,但不喜欢简单的翻译,而偏好对多篇文章的汇总或者点评,因此这件事就放下了。这篇小文龚鹤扬改了好几次,我问他后面的大段英文为何不翻译,他说这里的英文写的太漂亮了,怕翻译不好,因此就全文引用了,不过这对于巡洋舰的读者应该不是问题。
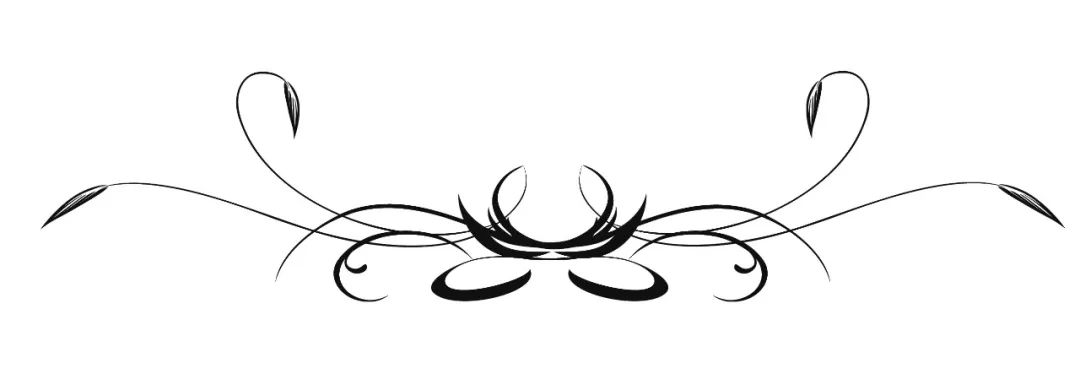
数据科学自古以来就是统计学的阵地, 然而这个阵地正在被机器学习蚕食。很多统计学家总是假定出一个模型,然后就说他们的模型怎么怎么好,但这是在很强的假定之下,比如数据一定要满足什么数学条件。一定要在这个条件下他们的模型才很好,他们最苦恼的就是寻找符合他们条件的数据(为了发表文章),但实际上只有老天爷才知道是否存在这样满足他们条件的数据(by 人大统计教授吴喜之)。统计学家总是在限制自我,Statistics are ruling out of all interesting questions(by Judea Pearl )。脱离实际的统计不但是无用的,而且是有害的,如今依然有很多教授在做着有害的统计学。
Leo Breiman,加州大学伯克利一个很有名的教授(CART决策树、bagging及随机森林发明者)是最早意识到经典统计学界问题的先驱者,故而在2001 写了一篇及其重要的文章《统计建模:两种文化》(Statistical Modeling: The Two Cultures)。该文章狠批了把数据限制在假定模型中的经典统计学界,然后大力推广他在商业咨询中用机器学习做算法模型的有效经验。涉及的两种文化包括:
这篇文章是机器学习和统计学在抢占数据科学这个阵地的第一篇高屋建瓴的深刻文章,影响了数据科学的发展路径。然而到今天将近20年过去了,机器学习取得了重大的胜利,甚至很多时候人们认为 AI 等于机器学习,而经典统计学中很有优秀的教授都已经行动了。
港大统计系系主任在2018年会上,呼吁系里面的老师用于拥抱AI。
美国两院院士统计学郁彬教授在去年在北大做报告的时候,批评北大统计系的老师眼里只有四大期刊,把自己圈子越做越少,呼吁新时代的统计学应该包括机器学习。
普林斯顿统计学教授范剑青今年刚刚发表第一篇关于 deep learning 的综述 on arxiv。
无数的机器学习应用已经落地,很显然,近20年来我们统计学的相关应用难以相提并论!我们数据模型文化并没有取得很大的进展!AlphaGo不是用数据模型,Deepfakes不是用数据模型,语音识别不是,人脸检测图像识别都不是。
机器学习,深度学习表面上大获全胜,然而却遇到了很多问题,于是有一种观点认为,AI应用的边界渐渐清晰,就是在语音和视觉领域。究竟遇到了什么困难呢?Pearl(2019) 认为是如下的三个困难:
这个三个困难是当前AI,当前数据科学的主要困难,科学家在尝试各种不同方法客服这些困难。Pearl(2019) The Seven Tools of Causal Inference, with Reflections on Machine Learning,这篇文曾经有过介绍让神经网络变得透明-因果推理对机器学习的八项助力
Pearl 提出解决现在的困境必须让机器学习因果推断,具体来说就是回答如下问题。
How can machines represent causal knowledge in a way that would enable them to access the necessary information swiftly, answer questions correctly, and do it with ease
这个问题在 Pearl的书籍《为什么》中被称做小图灵测试。当然有很多科学家针对AI面临的问题会有许多不同的解决方案,包括元学习,深度强化学习,规则学习知识图谱等等,但是个人觉得通过小图灵测试是众多方法构建强人工智能中最重要的一步。
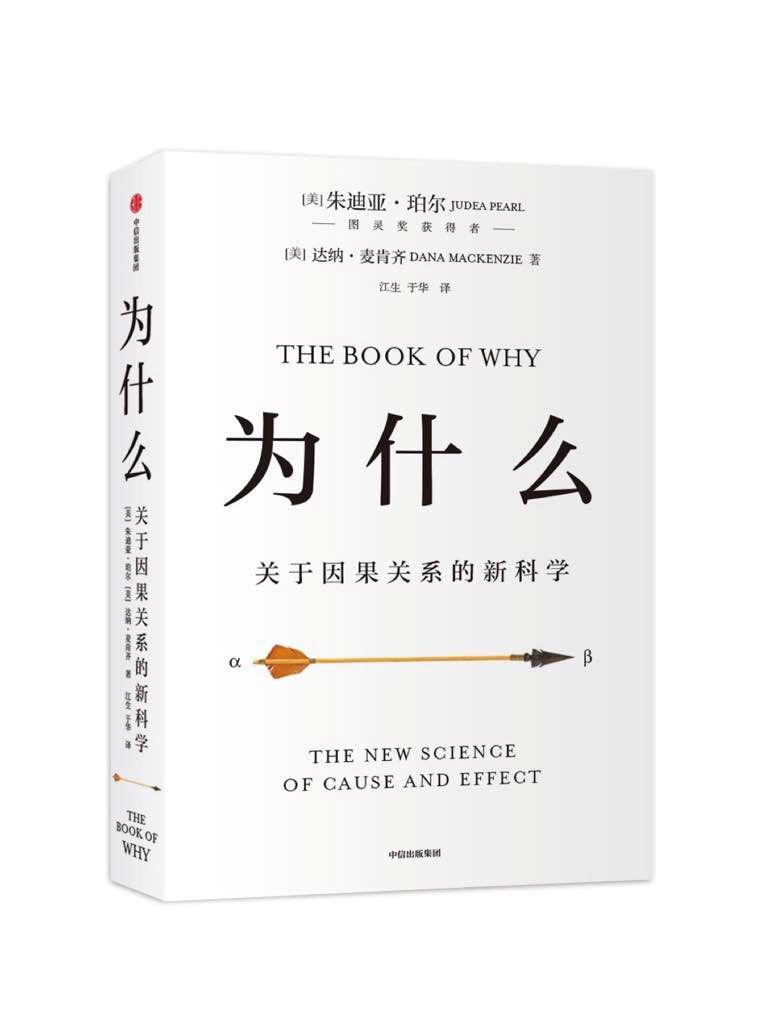
点击查看该书介绍从相关性到因果性-读《The book of why》以及
速读悖论,兼谈因果推断的重要性
Hernan(2019) 认为我们现在需要重新定义数据科学,需要因果推断放在数据科学的核心位置,数据科学的任务包括三类,描述,预测和反事实预测,具体来说:
Description is using data to provide a quantitative summary of certain features of the world.
Prediction is using data to map some features of the world to other features of the world.
Counterfactual prediction is using data to predict certain features of the world as if the world had been different, which is required in causal inference applications.
对于很多人来说,什么叫做反事实预测呢?
简单来说就是回答这样的问题:如果当初我 。。。, 那么现在会怎么样?如果我天天锻炼,以后会怎么样?Pearl 总结就是 “what if" kind of questions. 学术一点来说就是干预(interventional)问题和反思问题(retrospective or explanatory):X=x" role="presentation" style=" box-sizing: border-box; display: inline-table; line-height: normal; word-spacing: normal; overflow-wrap: normal; float: none; direction: ltr; max-width: none; max-height: none; min-width: 0px; min-height: 0px; border-width: 0px; border-style: initial; border-color: initial; ">what if I had been acted differently?
Hernan(2019) 中最后的结论是:
Data science is a component of many sciences, including the health and social ones. Therefore, the tasks of data science are the tasks of those sciences—description, prediction, causal inference. A sometimes-overlooked point is that a successful data science requires not only good data and algorithms, but also domain knowledge (including causal knowledge) from its parent sciences.
The current rebirth of data science is an opportunity to rethink data analysis free of the historical constraints imposed by traditional statistics, which have left scientists ill-equipped to handle causal questions. While the clout of statistics in scientific training and publishing impeded the introduction of a unified formal framework for causal inference in data analysis, the coining of the term “data science” and the recent influx of “data scientists” interested in causal analyses provides a once-in-a-generation chance of integrating all scientific questions, including causal ones, in a principled data analysis framework. An integrated data science curriculum can present a coherent conceptual framework that fosters understanding and collaboration between data analysts and domain experts.
更多阅读
因果推理入门指南-必须的7个步骤
参考文献
Breiman(2001) Statistical Modeling: The Two Cultures
Jianqing Fan(2019) A Selective Overview of Deep Learning https://arxiv.org/abs/1904.05526
Pearl(2019) The Seven Tools of Causal Inference, with Reflections on Machine Learning
Hernan(2019) A Second Chance to Get Causal Inference Right: A Classification of Data Science Tasks