原创内容第811篇,专注量化投资、个人成长与财富自由。今天再发布一个“会员专属”的策略,是个人比较喜欢的。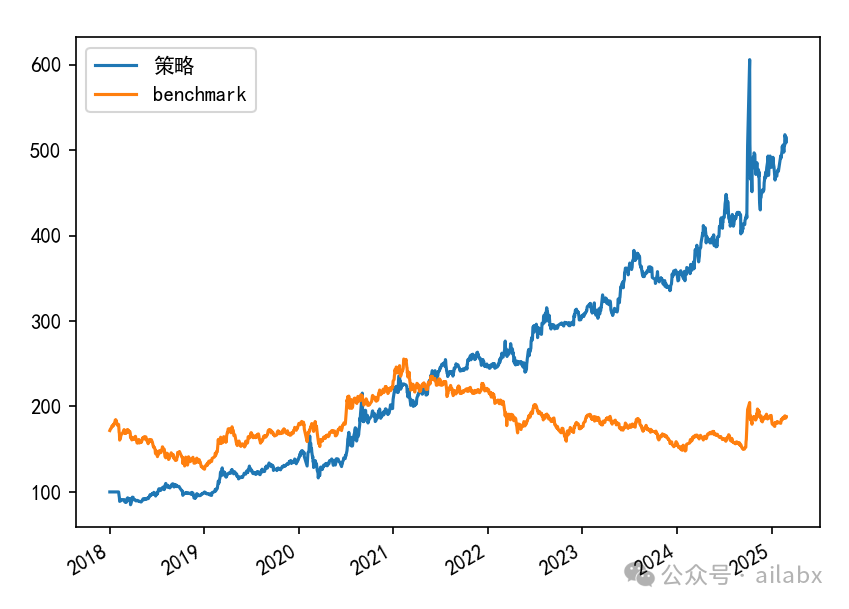
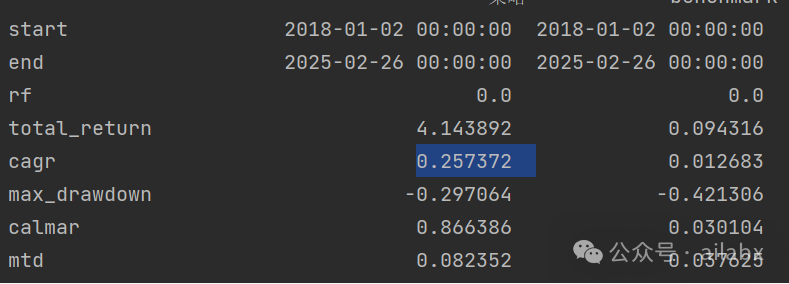
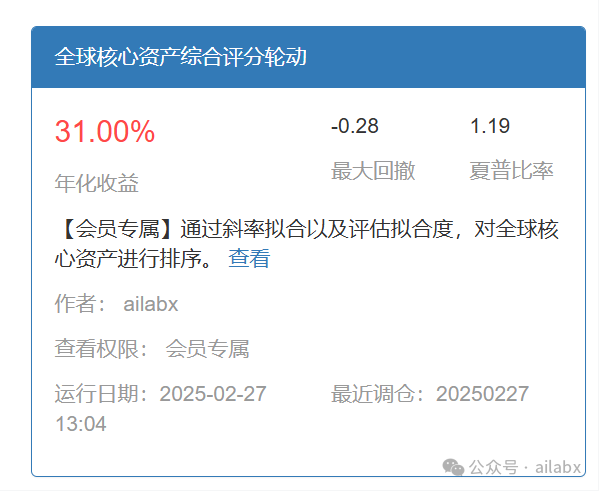
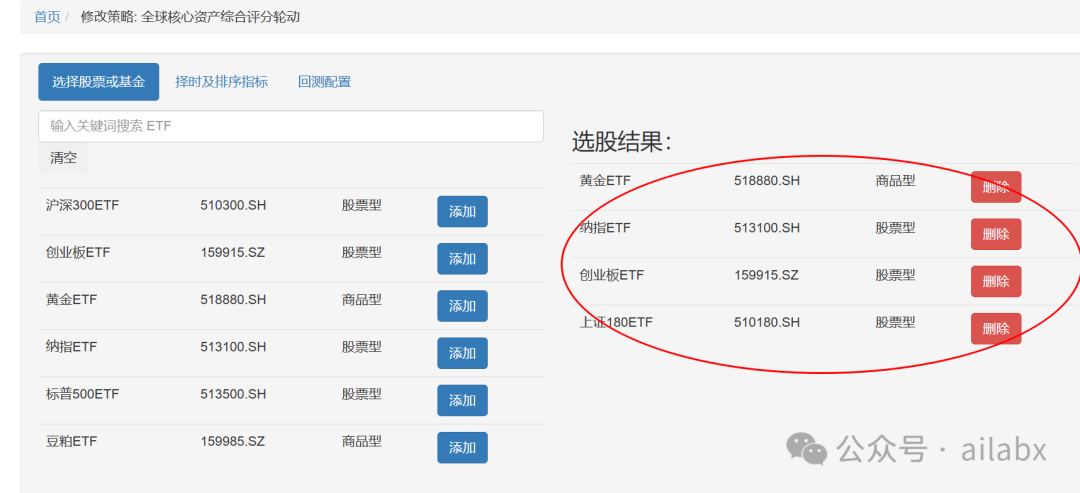
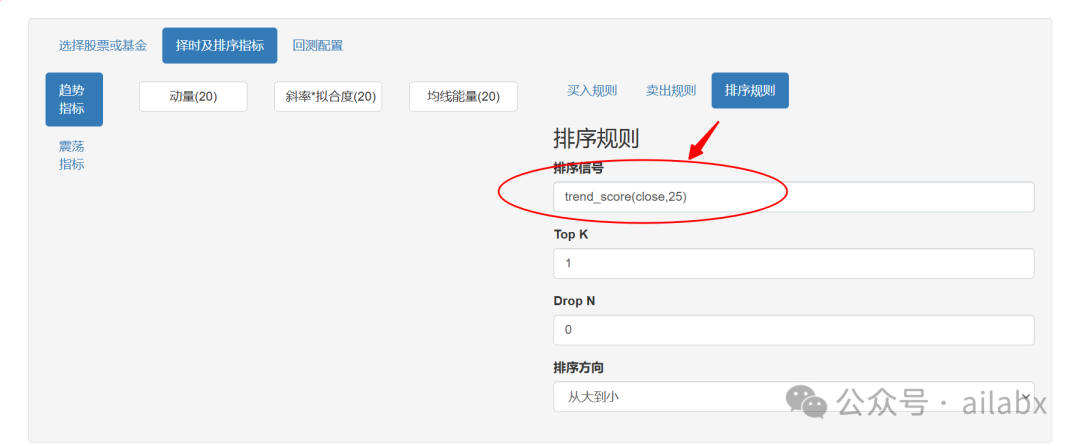
@calc_by_symbol
def trend_score(close, period=25):
"""
向量化计算趋势评分:年化收益率 × R平方
:param close: 收盘价序列(np.array或pd.Series)
:param period: 计算窗口长度,默认25天
:return: 趋势评分数组,长度与输入相同,前period-1位为NaN
"""
if len(close) < period:
return np.full_like(close, np.nan)
y = np.log(close)
windows = np.lib.stride_tricks.sliding_window_view(y, window_shape=period)
x = np.arange(period)
# 预计算固定值
n = period
sum_x = x.sum()
sum_x2 = (x ** 2).sum()
denominator = n * sum_x2 - sum_x ** 2
# 滑动窗口统计量
sum_y = windows.sum(axis=1)
sum_xy = (windows * x).sum(
axis=1)
# 回归系数
slope = (n * sum_xy - sum_x * sum_y) / denominator
intercept = (sum_y - slope * sum_x) / n
# 年化收益率
annualized_returns = np.exp(slope * 250) - 1
# R平方计算
y_pred = slope[:, None] * x + intercept[:, None]
residuals = windows - y_pred
ss_res = np.sum(residuals ** 2, axis=1)
sum_y2 = np.sum(windows ** 2, axis=1)
ss_tot = sum_y2 - (sum_y ** 2) / n
r_squared = 1 - (ss_res / ss_tot)
r_squared = np.nan_to_num(r_squared, nan=0.0) # 处理零方差情况
# 综合评分
score = annualized_returns * r_squared
# 对齐原始序列长度
full_score = np.full_like(y, np.nan)
full_score = pd.Series(index=close.index)
full_score[period - 1:] = score
return full_score
from bt_algos_extend import Task, Engine
def ranking_ETFs():
t = Task()
t.name = '基于ETF历史评分的轮动策略'
# 排序
t.period = 'RunDaily'
t.weight = 'WeighEqually'
t.order_by_signal = 'trend_score(close,25)'
t.start_date = '20180101'
#t.end_date = '20240501'
t.symbols = [
'518880.SH', # 黄金ETF(大宗商品)
'513100.SH', # 纳指100(海外资产)
'159915.SZ', # 创业板100(成长股,科技股,中小盘)
'510180.SH', # 上证180(价值股,蓝筹股,中大盘)
]
t.benchmark = '510300.SH'
return t
res = Engine().run(ranking_ETFs())
import matplotlib.pyplot as plt
print(res.stats)
from matplotlib import rcParams
rcParams['font.family'] = 'SimHei'
#res.plot_weights()
res.prices.plot()
plt.show()
AI量化实验室 星球,已经运行三年多,1500+会员。
aitrader代码,因子表达式引擎、遗传算法(Deap)因子挖掘引擎等,支持vnpy,qlib,backtrader和bt引擎,内置多个年化30%+的策略,每周五迭代一次,代码和数据在星球全部开源。