“ 快速高效学会python的终极奥义:过、抄、仿、改、调、看、练、创、悟”
正文开始前,首先声明下,我也是半路出家非科班出身,所以可能代码的规范
上一课我们大概学习了python的基本代码,最后一个部分,我们提到python相比于其他的编程语言,最实用也是最有魅力的地方就在于你可以找到各种各样的包包,他们几乎可以满足你所有的需求,非常适合不喜欢自己造轮子,喜欢抄代码的人比如我,如果你有一些定制化的需求,代码也都是开源的,可以自己扒拉下来再手动做一些修改,大大节约了工作量。
开始之前你需要了解的
今天主要介绍的就是做数据分析必不可少的一个包包,pandas,这里给大家吐血安利youtube上的一系列视频,是我自己觉得在市面上翻出来最实用的一套课,每个短片都控制在10分钟之内,想要真正学好的可以大致看一看,如果想偷懒,可以直接看我的精华提炼版本,然后代码也不用都记住,只要用的时候翻到我的帖子,然后全文搜索下,再ctrl C大法好粘贴过去,我把视频里面提到的方法全都汇总在下面了,方便大家copy~~~
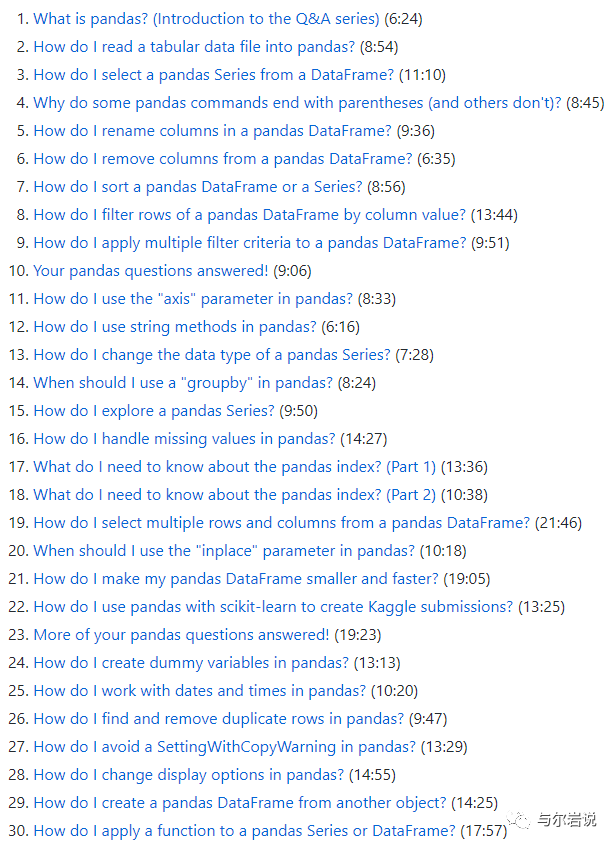
微信没办法粘贴外链过来,就直接手动贴一个,其他都在这个目录底下~
https://www.youtube.com/watch?v=yzIMircGU5I&list=PL5-da3qGB5ICCsgW1MxlZ0Hq8LL5U3u9y&index=2
https://pandas.pydata.org/docs/user_guide/index.html#user-guide
跟上节课一样,我还是习惯先肢解下课程内容,其实pandas你就想成学excel就好,excel的基本操作有什么,pandas就基本有什么,那excel的功能大致上有:数据转换(导入各种文件类型,生成各种文件类型)、表格建立和编辑(包括绝对位置和相对位置的取数、增删行、列,两个表格横着竖着拼接等等)、数据分析和处理(包括数据透视表、各种函数、画各种图表),pandas的功能也基本就是这些,有更细致的需求,直接百度或者在我的文章里关键词检索,然后copy代码就可以,基本的操作只需要掌握这些。照例有需要的朋友可以转发+后台留言,我给大家发课后的代码,可以自己再练习~~
基本内容学习
第一步永远是导入包包
from pandas import Series, DataFrame
import pandas as pd
参考:
https://amaozhao.gitbooks.io/pandas-notebook/content/
https://github.com/justmarkham/pandas-videos/blob/master/pandas.ipynb
pandas的数据类型
有两类,一种是dataframe一种是series,其中dataframe是多行多列,而series是单列多行,其都有行和列组成。
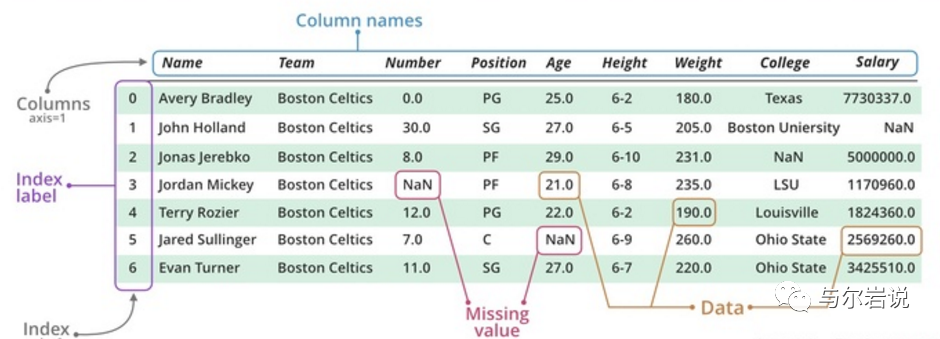
创建series和dataframe
1.创建series
通过列表创建
obj = Series([4, 7, -5, 3])
obj2 = Series([4, 7, -5, 3], index=['d','b','a','c']) #指定索引
通过字典创建
sdata = {'Ohio':35000, 'Texas':7100, 'Oregon':1600,'Utah':500}
obj3 = Series(sdata)
通过字典加索引
states = ['California', 'Ohio', 'Oregon', 'Texas']
obj4 = Series(sdata, index=states)
2.创建dataframe
词典生成
data = {'state':['Ohio', 'Ohio', 'Ohio', 'Nevada','Nevada'],
'year':[2000, 2001, 2002, 2011, 2002],
'pop':[1.5, 1.7, 3.6, 2.4, 2.9]}
frame = DataFrame(data)
frame2 = DataFrame(data, columns=['year', 'state', 'pop']) #指定列
frame3 = DataFrame(data, columns=['year', 'state', 'pop'],
index=['one', 'two', 'three', 'four', 'five']) #指定列和索引
列表生成(常用)
errors = [('c',1,'right'), ('b', 2,'wrong')]
df = pd.DataFrame(errors,columns=['name', 'count', 'result'])
嵌套词典
pop = {'Nevada':{2001:2.4, 2002:2.9},
'Ohio':{2000:1.5, 2001:1.7, 2002
:3.6}}
frame4 = DataFrame(pop)
Out[138]:
Nevada Ohio
2000 NaN 1.5
2001 2.4 1.7
2002 2.9 3.6
series组合
In [4]: a = pd.Series([1,2,3])
In [5]: b = pd.Series([2,3,4])
In [6]: c = pd.DataFrame([a,b])
In [7]: c
Out[7]:
0 1 2
0 1 2 3
1 2 3 4
#或者也可以按照列来生成dataframe
c = pd.DataFrame({'a':a,'b':b})
读取数据
pandas可以读取多种数据类型,包括但是不限于csv、txt、xlsx、json、html、sql等等,只有你想不到没有他不能读,一般读取通过read_xxx输出通过to_xxx
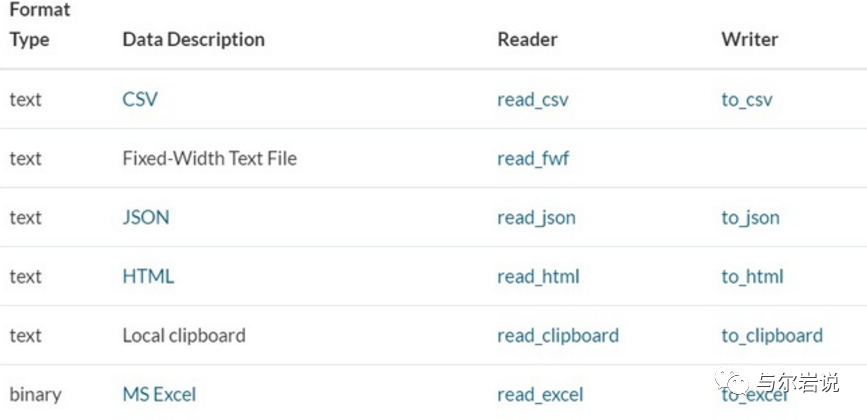
索引和选取
对于一组数据:
data = DataFrame(np.arange(16).reshape((4,4)),index=['Ohio', 'Colorado','Utah','New York'],columns=['one','two','three','four'])
>>> data
one two three four
Ohio 0 1 2 3
Colorado 4 5 6 7
Utah 8 9 10 11
New York 12 13 14 15
几种索引方式
事实上有很多种索引方式,ix是较老的方式
data['two']
data.ix['Ohio']
data.ix['Ohio', ['two','three']]
较新的是loc与iloc
loc用于索引中有文字的,iloc仅仅用于索引为数字的
然后比较坑的是经常是索引不成功,
对于列来说:这时候要检查下这个名字对不对?可以用
data.columns=[]
来强行规定名字然后再索引
对于行来说:可以强行reset_index或者set_index为新的一组值,基本可以避免大坑
按照索引来遍历
按照行来进行,这个操作其实很低效率
lbsdata=pd.read_csv('testlocation.csv',sep='\t')
lbstagRow = pd.DataFrame()
lbstag = pd.DataFrame()
for
index, row in lbsdata.iterrows():
lbstagRow['recommend'] = [jsonToSp['formatted_address']]
lbstagRow['city'] = [jsonToSparse['addressComponent']]
lbstag = lbstag.append(lbstagRow,ignore_index=True)
也可以:
for ix, row in df.iterrows():
for ix, col in df.iteritems():
for i in range(0,len(df)):
排序
按列排序
In[73]: obj = Series(range(4), index=['d','a','b','c'])
In[74]: obj.sort_index()
Out[74]:
a 1
b 2
c 3
d 0
dtype: int64
In[78]: frame = DataFrame(np.arange(8).reshape((2,4)),index=['three', 'one'],columns=['d','a','b','c'])
In[79]: frame
Out[79]:
d a b c
three 0 1 2 3
one 4 5 6 7
In[86]: frame.sort_index()
Out[86]:
d a b c
one 4 5 6 7
three 0 1 2 3
In[87]: frame.sort()
Out[87]:
d a b c
one 4 5 6 7
three 0 1 2 3
按行排序
In[89]: frame.sort_index(axis=1, ascending=False)
Out[89]:
d c b a
three 0 3 2 1
one 4 7 6 5
按值排序
frame.sort_values('a')
删除
删除指定轴上的项
即删除 Series 的元素或 DataFrame 的某一行(列)的意思,通过对象的 .drop(labels, axis=0) 方法:
In[11]: ser = Series([4.5,7.2,-5.3,3.6], index=['d','b','a','c'])
In[13]: ser.drop('c')
Out[13]:
d 4.5
b 7.2
a -5.3
dtype: float64
删除行和列
In[17]: df = DataFrame(np.arange(9).reshape(3,3), index=['a','c','d'], columns=['oh','te','ca'])
In[18]: df
Out[18]:
oh te ca
a 0 1 2
c 3 4 5
d 6 7 8
In[19]: df.drop('a')
Out[19]:
oh te ca
c 3 4 5
d 6 7 8
In[20]: df.drop(['oh','te'],axis=1)
Out[20]:
ca
a 2
c 5
d 8
删除点
df_train.sort_values(by = 'GrLivArea',ascending = False)[:2]
df_train = df_train.drop(df_train[df_train['Id'] == 1299].index)
df_train = df_train.drop(df_train[df_train['Id'] == 524].index)
DataFrame连接
算术运算
In[5]: df1 = DataFrame(np.arange(12.).reshape((3,4)),columns=list('abcd'))
In[6]: df2 = DataFrame(np.arange(20.).reshape((4,5)),columns=list('abcde'))
In[9]: df1+df2
Out[9]:
a b c d e
0 0 2 4 6 NaN
1 9 11 13 15 NaN
2 18 20 22 24 NaN
3 NaN NaN NaN NaN NaN
# 传入填充值
In[11]: df1.add(df2, fill_value=0)
Out[11]:
a b c d e
0 0 2 4 6 4
1 9 11 13 15 9
2 18 20 22 24 14
3 15 16 17 18 19
merge
pandas.merge可根据一个或多个键将不同DataFrame中的行连接起来。
默认情况下,merge做的是“inner”连接,结果中的键是交集,其它方式还有“left”,“right”,“outer”。“outer”外连接求取的是键的并集,组合了左连接和右连接。
In[14]: df1 = DataFrame({'key':['b','b','a','c','a','a','b'],'data1':range(7)})
In[15]: df2 = DataFrame({'key':['a','b','d'],'data2':range(3)})
In[18]: pd.merge(df1, df2) #或显式: pd.merge(df1, df2, on='key')
Out[18]:
data1 key data2
0 0 b 1
1 1 b 1
2 6 b 1
3 2 a 0
4 4 a 0
5 5 a 0
concat
这种数据合并运算被称为连接(concatenation)、绑定(binding)或堆叠(stacking)。默认情况下,concat是在axis=0(行)上工作的,最终产生一个新的Series。如果传入axis=1(列),则变成一个DataFrame。
pd.concat([s1,s2,s3], axis=1)
dfs = []
for classify in classify_finance + classify_other:
sql = "select classify, tags from {} where classify='{}' length(tags)>0 limit 1000".format(mysql_table_sina_news_all, classify)
df = pd.read_sql(sql,engine)
dfs.append(df)
df_all = pd.concat(dfs, ignore_index=True)
数据转换
数据过滤、清理以及其他的转换工作。
移除重复数据(去重)
duplicated()
DataFrame的duplicated方法返回一个布尔型Series,表示各行是否是重复行
df = DataFrame({'k1':['one']*3 + ['two']*4, 'k2':[1,1,2,3,3,4,4]})
df.duplicated()
df.drop_duplicates()
利用函数或映射进行数据转换
对于数据:
df = DataFrame({'food':['bacon','pulled pork','bacon','Pastraml','corned beef', 'Bacon', 'pastraml','honey ham','nova lox'],'ounces':[4,3,12,6,7.5,8,3,5,6]})
增加一列表示该肉类食物来源的动物类型,先编写一个肉类到动物的映射:
meat_to_animal = {'bacon':'pig',
'pulled pork':'pig',
'pastraml':'cow',
'corned beef':'cow',
'honey ham':'pig',
'nova lox':'salmon'}
map
Series的map方法可以接受一个函数或含有映射关系的字典型对象。
df['animal'] = df['food'].map(str.lower).map(meat_to_animal)
df['food'].map(lambda x : meat_to_animal[x.lower()])
Out[22]:
0 pig
1 pig
2 pig
3 cow
4 cow
5 pig
6 cow
7 pig
8 salmon
apply 和 applymap
对于DataFrame:
In[21]: df = DataFrame(np.random.randn(4,3), columns=list('bde'),index=['Utah','Ohio','Texas','Oregon'])
In[22]: df
Out[22]:
b d e
Utah 1.654850 0.594738 -1.969539
Ohio 2.178748 1.127218 0.451690
Texas 1.209098 -0.604432 -1.178433
Oregon 0.286382
0.042102 -0.345722
apply将函数应用到由各列或行所形成的一维数组上。
作用到列:
In[24]: f = lambda x : x.max() - x.min()
In[25]: df.apply(f)
Out[25]:
b 1.892366
d 1.731650
e 2.421229
dtype: float64
作用到行
In[26]: df.apply(f, axis=1)
Out[26]:
Utah 3.624390
Ohio 1.727058
Texas 2.387531
Oregon 0.632104
dtype: float64
作用到每个元素
In[70]: frame = DataFrame(np.random.randn(4,3), columns=list('bde'),index=['Utah','Ohio','Texas','Oregon'])
In[72]: frame.applymap(lambda x : '%.2f' % x)
Out[72]:
b d e
Utah 1.19 1.56 -1.13
Ohio 0.10 -1.03 -0.04
Texas -0.22 0.77 -0.73
Oregon 0.22 -2.06 -1.25
numpy的ufuncs
Numpy的ufuncs(元素级数组方法)也可用于操作pandas对象。
取绝对值操作np.abs(),np.max(),np.min()
替换值
In[23]: se = Series([1, -999, 2, -999, -1000, 3])
In[24]: se.replace(-999, np.nan)
Out[24]:
0 1
1 NaN
2 2
3 NaN
4 -1000
5 3
dtype: float64
In[25]: se.replace([-999, -1000], np.nan)
Out[25]:
0 1
1 NaN
2 2
3 NaN
4 NaN
5 3
dtype: float64
In[26]: se.replace([-999, -1000], [np.nan, 0])
Out[26]:
0 1
1 NaN
2 2
3 NaN
4 0
5 3
dtype: float64
# 字典
In[27]: se.replace({-999:np.nan, -1000:0})
Out[27]:
0 1
1 NaN
2 2
3 NaN
4 0
5 3
dtype: float64
df.rename(index=str.title, columns=str.upper)
df.rename(index={'OHIO':'INDIANA'}, columns={'three':'peekaboo'})
离散化和面元划分
pd.cut
为了便于分析,连续数据常常离散化或拆分为“面元”(bin)。比如:
ages = [20, 22,25,27,21,23,37,31,61,45,41,32]
需要将其划分为“18到25”, “26到35”,“36到60”以及“60以上”几个面元。要实现该功能,需要使用pandas的cut函数。
bins = [18, 25, 35, 60, 100]
cats = pd.cut(ages, bins)
Out[39]:
[(18, 25], (18, 25], (18, 25], (25, 35], (18, 25], ..., (25, 35], (60, 100], (35, 60], (35, 60], (25, 35]]
Length: 12
Categories (4, object): [(18, 25] < (25, 35] < (35, 60] < (60, 100]]
可以通过right=False指定哪端是开区间或闭区间。也可以指定面元的名称:
cats = pd.cut(ages, bins, right=False)
group_name = ['Youth', 'YoungAdult', 'MiddleAged', 'Senior']
cats = pd.cut(ages, bins, labels=group_name)
pd.value_counts(cats)
Out[46]:
Youth 5
MiddleAged 3
YoungAdult 3
Senior 1
dtype: int64
pd.qcut
qcut是一个非常类似cut的函数,它可以根据样本分位数对数据进行面元划分,根据数据的分布情况,cut可能无法使各个面元中含有相同数量的数据点,而qcut由于使用的是样本分位数,可以得到大小基本相等的面元。
In[48]: data = np.random.randn(1000)
In[49]: cats = pd.qcut(data, 4)
In[50]: cats
Out[50]:
[(0.577, 3.564], (-0.729, -0.0341], (-0.729, -0.0341], (0.577, 3.564], (0.577, 3.564], ..., [-3.0316, -0.729], [-3.0316, -0.729], (-0.0341, 0.577], [-3.0316, -0.729], (-0.0341, 0.577]]
Length: 1000
Categories (4, object): [[-3.0316, -0.729] < (-0.729, -0.0341] < (-0.0341, 0.577] < (0.577, 3.564]]
In[51]: pd.value_counts(cats)
Out[51]:
(0.577, 3.564] 250
(-0.0341, 0.577] 250
(-0.729, -0.0341] 250
[-3.0316, -0.729] 250
dtype: int64
检测和过滤异常值
可以先describe然后在找出超过某一个阈值的
data[(np.abs(data)>3).any(1)]
data[np.abs(data)>3] = np.sign(data)*3
In[63]: data.describe()
Out[63]:
0 1 2 3
count 1000.000000 1000.000000 1000.000000 1000.000000
mean -0.067623 0.068473 0.025153 -0.002081
std 0.995485 0.990253 1.003977 0.989736
min -3.000000 -3.000000 -3.000000 -3.000000
25% -0.774890 -0.591841 -0.641675 -0.644144
50% -0.116401 0.101143 0.002073 -0.013611
75% 0.616366 0.780282 0.680391 0.654328
max 3.000000 2.653656 3.000000 3.000000
单因素分析
这里的关键在于如何建立阈值,定义一个观察值为异常值。我们对数据进行正态化,意味着把数据值转换成均值为0,方差为1的数据。
saleprice_scaled= StandardScaler().fit_transform(df_train['SalePrice'][:,np.newaxis]);
low_range = saleprice_scaled[saleprice_scaled[:,0].argsort()][:10]
high_range= saleprice_scaled[saleprice_scaled[:,0].argsort()][-10:]
print('outer range (low) of the distribution:')
print(low_range)
print('\nouter range (high) of thedistribution:')
print(high_range)
进行正态化后,可以看出:
低范围的值都比较相似并且在0附近分布。
高范围的值离0很远,并且七点几的值远在正常范围之外。
双变量分析
'GrLivArea'和'SalePrice'双变量分析
var = 'GrLivArea'
data = pd.concat([df_train['SalePrice'], df_train[var]], axis=1)
data.plot.scatter(x=var, y='SalePrice', ylim=(0,800000));
相关性探索
与数字型变量的关系
var = 'GrLivArea'
data = pd.concat([df_train['SalePrice'], df_train[var]], axis=1)
data.plot.scatter(x=var, y='SalePrice', ylim=(0,800000));
与类别型变量的关系
var = 'OverallQual'
data = pd.concat([df_train['SalePrice'], df_train[var]], axis=1)
f, ax = plt.subplots(figsize=(8, 6))
fig = sns.boxplot(x=var, y="SalePrice", data=data)
fig.axis(ymin=0, ymax=800000);
相关性分析
相关系数矩阵
corrmat = df_train.corr()
f, ax = plt.subplots(figsize=(12, 9))
sns.heatmap(corrmat, vmax=.8, square=True);
最近的相关系数矩阵
k = 10 #number ofvariables for heatmap
cols = corrmat.nlargest(k, 'SalePrice')['SalePrice'].index
cm = np.corrcoef(df_train[cols].values.T)
sns.set(font_scale=1.25)
hm = sns.heatmap(cm, cbar=True, annot=True, square=True, fmt='.2f', annot_kws={'size': 10},
yticklabels=cols.values, xticklabels=cols.values)
plt.show()
两两之间的散点图
sns.set()
cols = ['SalePrice', 'OverallQual', 'GrLivArea','GarageCars', 'TotalBsmtSF', 'FullBath', 'YearBuilt']
sns.pairplot(df_train[cols], size = 2.5)
plt.show();
缺失数据
关于缺失数据需要思考的重要问题:
这一缺失数据的普遍性如何?
缺失数据是随机的还是有律可循?
这些问题的答案是很重要的,因为缺失数据意味着样本大小的缩减,这会阻止我们的分析进程。除此之外,以实质性的角度来说,我们需要保证对缺失数据的处理不会出现偏离或隐藏任何难以忽视的真相。
total= df_train.isnull().sum().sort_values(ascending=False)
percent = (df_train.isnull().sum()/df_train.isnull().count()).sort_values(ascending=False)
missing_data = pd.concat([total, percent], axis=1, keys=['Total','Percent'])
missing_data.head(20)
当超过15%的数据都缺失的时候,我们应该删掉相关变量且假设该变量并不存在。
根据这一条,一系列变量都应该删掉,例如'PoolQC', 'MiscFeature', 'Alley'等等,这些变量都不是很重要,因为他们基本都不是我们买房子时会考虑的因素。
df_train= df_train.drop((missing_data[missing_data['Total'] > 1]).index,1)
df_train= df_train.drop(df_train.loc[df_train['Electrical'].isnull()].index)
df_train.isnull().sum().max() #justchecking that there's no missing data missing..
多元分析
正态性:
应主要关注以下两点:
直方图 - 峰度和偏度。
正态概率图 - 数据分布应紧密跟随代表正态分布的对角线。
‘SalePrice’
绘制直方图和正态概率图:
sns.distplot(df_train['SalePrice'], fit=norm);
fig = plt.figure()
res = stats.probplot(df_train['SalePrice'], plot=plt)
可以看出,房价分布不是正态的,显示了峰值,正偏度,但是并不跟随对角线。
可以用对数变换来解决这个问题
进行对数变换:
df_train['SalePrice']= np.log(df_train['SalePrice'])
绘制变换后的直方图和正态概率图:
sns.distplot(df_train['SalePrice'], fit=norm);
fig = plt.figure()
res = stats.probplot(df_train['SalePrice'], plot=plt)
同方差性:
最好的测量两个变量的同方差性的方法就是图像。
'SalePrice' 和 'GrLivArea'同方差性
绘制散点图:
plt.scatter(df_train['GrLivArea'],df_train['SalePrice']);
虚拟变量
将类别变量转换为虚拟变量:
df_train = pd.get_dummies(df_train)
def factor(series):
levels = list(set(series))
design_matrix = np.zeros((len(series), len(levels)))
for row_index, elem in enumerate(series):
design_matrix[row_index, levels.index(elem)] = 1
name = series.name or ""
columns = map(lambda level: "%s[%s]" % (name, level), levels)
df = pd.DataFrame(design_matrix, index=series.index,
columns=columns)
return df
在处理类别变量的时候可以:
data.apply(lambda x: sum(x.isnull()))
var = ['Gender','Salary_Account','Mobile_Verified','Var1','Filled_Form','Device_Type','Var2','Source']
for v in var:
print '\nFrequency count for variable %s'%v
print data[v].value_counts()
print len(data['City'].unique())
#drop city because too many unique
data.drop('City',axis=1,inplace=True)
更加简单的方法是:
from sklearn.preprocessing import LabelEncoder
le = LabelEncoder()
var_to_encode = ['Device_Type','Filled_Form','Gender','Var1','Var2','Mobile_Verified','Source']
for col in var_to_encode:
data[col] = le.fit_transform(data[col])
data = pd.get_dummies(data, columns=var_to_encode)
data.columns
处理时间变量
data['Age'] = data['DOB'].apply(lambda x: 115 - int(x[-2:]))
data['Age'].head()
df_train.onlineDate=pd.to_datetime(df_train.onlineDate)
df_train['age']=(datetime.date.today()-df_train.onlineDate).dt.days
词向量化
# import and init CountVectorizer
from sklearn.feature_extraction.text import CountVectorizer
vectorizer = CountVectorizer()
vectorizer # default parameters
CountVectorizer(analyzer='word', binary=False, decode_error='strict',
dtype='numpy.int64'>, encoding='utf-8', input='content',
lowercase=True, max_df=1.0, max_features=None, min_df=1,
ngram_range=(1, 1), preprocessor=None, stop_words=None,
strip_accents=None, token_pattern='(?u)\\b\\w\\w+\\b',
tokenizer=None, vocabulary=None)
# transform text into document-term matrix
X = vectorizer.fit_transform(reviews)
print(type(X)) # X is a sparse matrix
print(X)
处理百分号
p_float = df['p_str'].str.strip("%").astype(float)/100;
p_float_2 = p_float.round(decimals=2)
p_str_2 = p_float.apply(lambda x: format(x, '.2%'));
特征离散化
s_amount_C = df_lc_C['借款金额']
q = [0.05, 0.2, 0.5, 0.8, 0.95]
bins = s_amount_C.quantile(q)
s_fea_dct_amount_C = pd.cut(s_amount_C, add_ends_to_bins(bins.values))
dict_s_fea_dct['amount'] = s_fea_dct_amount_C
s_term_C = df_lc_C['借款期限']
q = [0.05, 0.2, 0.5, 0.8, 0.95]
bins = s_term_C.quantile(q)
sns.countplot(s_term_C);
看有多少个类别
def add_ends_to_bins(bins):
return np.append(np.insert(bins, 0, -np.inf), np.inf)
df_lc_C.groupby(s_type_C).size()
for col in category:
print(df_train2[str(col)].value_counts(dropna=False))
sns.countplot(s_is_first_C);
处理不同类别变量
DataFrame.convert_objects(convert_dates=True, convert_numeric=False, convert_timedeltas=True, copy=True)
这个命令非常好用!
使效率倍增的Pandas使用技巧
本文取自Analytics Vidhya的一个帖子12 Useful Pandas Techniques in Python for Data Manipulation,浏览原帖可直接点击链接,中文版可参见Datartisan的用 Python 做数据处理必看:12 个使效率倍增的 Pandas 技巧。这里主要对帖子内容进行检验并记录有用的知识点。
数据集
首先这个帖子用到的数据集是datahack的贷款预测(load prediction)竞赛数据集,点击链接可以访问下载页面,如果失效只需要注册后搜索loan prediction竞赛就可以找到了。入坑的感兴趣的同学可以前往下载数据集,我就不传上来github了。
先简单看一看数据集结构(此处表格可左右拖动):
Loan_ID | Gender | Married | Dependents | Education | Self_Employed | ApplicantIncome | CoapplicantIncome | LoanAmount | Loan_Amount_Term | Credit_History | Property_Area | Loan_Status |
---|
LP001002 | Male | No | 0 | Graduate | No | 5849 | 0 |
| 360 | 1 | Urban | Y |
LP001003 | Male | Yes | 1 | Graduate | No | 4583 | 1508 | 128 | 360 | 1 | Rural | N |
LP001005 | Male | Yes | 0 | Graduate | Yes |
3000 | 0 | 66 | 360 | 1 | Urban | Y |
LP001006 | Male | Yes | 0 | Not Graduate | No | 2583 | 2358 | 120 | 360 | 1 | Urban | Y |
LP001008 | Male | No | 0 | Graduate | No | 6000 | 0 | 141 | 360 | 1 | Urban | Y |
共614条数据记录,每条记录有十二个基本属性,一个目标属性(Loan_Status)。数据记录可能包含缺失值。
数据集很小,直接导入Python环境即可:
import pandas as pd
import numpy as np
data = pd.read_csv(r"F:\Datahack_Loan_Prediction\Loan_Prediction_Train.csv", index_col="Loan_ID")
注意这里我们指定index_col为Loan_ID这一列,所以程序会把csv文件中Loan_ID这一列作为DataFrame的索引。默认情况下index_col为False,程序自动创建int型索引,从0开始。
1. 布尔索引(Boolean Indexing)
有时我们希望基于某些列的条件筛选出需要的记录,这时可以使用loc索引的布尔索引功能。比方说下面的代码筛选出全部无大学学历但有贷款的女性列表:
data.loc[(data["Gender"]=="Female") & (data["Education"]=="Not Graduate") & (data["Loan_Status"]=="Y"), ["Gender","Education","Loan_Status"]]
loc索引是优先采用label定位的,label可以理解为索引。前面我们定义了Loan_ID为索引,所以对这个DataFrame使用loc定位时就可以用Loan_ID定位:
data.loc['LP001002']
Gender Male
Married No
Dependents 0
Education Graduate
Self_Employed No
ApplicantIncome 5849
CoapplicantIncome 0
LoanAmount 129.937
Loan_Amount_Term 360
Credit_History 1
Property_Area Urban
Loan_Status Y
LoanAmount_Bin medium
Loan_Status_Coded 1
Name: LP001002, dtype: object
可以看到我们指定了一个Loan_ID,就定位到这个Loan_ID对应的记录。
loc允许四种input方式:
指定一个label;
label列表,比如['LP001002','LP001003','LP001004']
;
label切片,比如'LP001002':'LP001003'
;
布尔数组
我们希望基于列值进行筛选就用到了第4种input方式-布尔索引。使用()括起筛选条件,多个筛选条件之间使用逻辑运算符&
,|
,~
与或非进行连接,特别注意,和我们平常使用Python不同,这里用and
,or
,not
是行不通的。
此外,这四种input方式都支持第二个参数,使用一个columns的列表,表示只取出记录中的某些列。上面的例子就是只取出了Gender
,Education
,Loan_Status
这三列,当然,获得的新DataFrame的索引依然是Loan_ID。
想了解更多请阅读 Pandas Selecting and Indexing。
2. Apply函数
Apply是一个方便我们处理数据的函数,可以把我们指定的一个函数应用到DataFrame的每一行或者每一列(使用参数axis设定,默认为0,即应用到每一列)。
如果要应用到特定的行和列只需要先提取出来再apply就可以了,比如data['Gender'].apply(func)
。特别地,这里指定的函数可以是系统自带的,也可以是我们定义的(可以用匿名函数)。比如下面这个例子统计每一列的缺失值个数:
# 创建一个新函数:
def num_missing(x):
return sum(x.isnull())
# Apply到每一列:
print("Missing values per column:")
print(data.apply(num_missing, axis=0)) # axis=0代表函数应用于每一列
Missing values per column:
Gender 13
Married 3
Dependents 15
Education 0
Self_Employed 32
ApplicantIncome 0
CoapplicantIncome 0
LoanAmount 22
Loan_Amount_Term 14
Credit_History 50
Property_Area 0
Loan_Status 0
dtype: int64
下面这个例子统计每一行的缺失值个数,因为行数太多,所以使用head函数仅打印出DataFrame的前5行:
# Apply到每一行:
print("\nMissing values per row:")
print(data.apply(num_missing, axis=1).head()) # axis=1代表函数应用于每一行
Missing values per row:
Loan_ID
LP001002 1
LP001003 0
LP001005 0
LP001006 0
LP001008 0
dtype: int64
想了解更多请阅读 Pandas Reference (apply)
3. 替换缺失值
一般来说我们会把某一列的缺失值替换为所在列的平均值/众数/中位数。fillna()
函数可以帮我们实现这个功能。但首先要从scipy库导入获取统计值的函数。
# 首先导入一个寻找众数的函数:
from scipy.stats import mode
GenderMode = mode(data['Gender'].dropna())
print(GenderMode)
print(GenderMode.mode[0],':',GenderMode.count[0])
ModeResult(mode=array(['Male'], dtype=object), count=array([489]))
Male : 489
F:\Anaconda3\lib\site-packages\scipy\stats\stats.py:257: RuntimeWarning: The input array could not be properly checked for nan values. nan values will be ignored.
"values. nan values will be ignored.", RuntimeWarning)
可以看到对DataFrame的某一列使用mode函数可以得到这一列的众数以及它所出现的次数,由于众数可能不止一个,所以众数的结果是一个列表,对应地出现次数也是一个列表。可以使用.mode
和.count
提取出这两个列表。
特别留意,可能是版本原因,我使用的scipy (0.17.0)不支持原帖子中的代码,直接使用mode(data['Gender'])
是会报错的:
F:\Anaconda3\lib\site-packages\scipy\stats\stats.py:257: RuntimeWarning: The input array could not be p
roperly checked for nan values. nan values will be ignored.
"values. nan values will be ignored.", RuntimeWarning)
Traceback (most recent call last):
File "", line 1, in
File "F:\Anaconda3\lib\site-packages\scipy\stats\stats.py", line 644, in mode
scores = np.unique(np.ravel(a)) # get ALL unique values
File "F:\Anaconda3\lib\site-packages\numpy\lib\arraysetops.py", line 198, in unique
ar.sort()
TypeError: unorderable types: str() > float()
必须使用mode(data['Gender'].dropna())
,传入dropna()处理后的DataFrame才可以。虽然Warning仍然存在,但是可以执行得到结果。Stackoverflow里有这个问题的讨论:Scipy Stats Mode function gives Type Error unorderable types。指出scipy的mode函数无法处理列表中包含混合类型的情况,比方说上面的例子就是包含了缺失值NAN类型和字符串类型,所以无法直接处理。
同时也指出Pandas自带的mode函数是可以处理混合类型的,我测试了一下:
from pandas import Series
Series.mode(data['Gender'])
0 Male
dtype: object
确实没问题,不需要使用dropna()处理,但是只能获得众数,没有对应的出现次数。返回结果是一个Pandas的Series对象。可以有多个众数,索引是int类型,从0开始。
掌握了获取众数的方法后就可以使用fiilna替换缺失值了:
#值替换:
data['Gender'].fillna(GenderMode.mode[0], inplace=True)
MarriedMode = mode(data['Married'].dropna())
data['Married'].fillna(MarriedMode.mode[0], inplace=True)
Self_EmployedMode = mode(data['Self_Employed'].dropna())
data['Self_Employed'].fillna(Self_EmployedMode.mode[0], inplace=True)
先提取出某一列,然后用fillna把这一列的缺失值都替换为计算好的平均值/众数/中位数。inplace关键字用于指定是否直接对这个对象进行修改,默认是False,如果指定为True则直接在对象上进行修改,其他地方调用这个对象时也会收到影响。这里我们希望修改直接覆盖缺失值,所以指定为True。
#再次检查缺失值以确认:
print(data.apply(num_missing, axis=0))
Gender 0
Married 0
Dependents 15
Education
0
Self_Employed 0
ApplicantIncome 0
CoapplicantIncome 0
LoanAmount 22
Loan_Amount_Term 14
Credit_History 50
Property_Area 0
Loan_Status 0
dtype: int64
可以看到Gender
,Married
,Self_Employed
这几列的缺失值已经都替换成功了,所以缺失值的个数为0。
想了解更多请阅读 Pandas Reference (fillna)
4. 透视表
透视表(Pivot Table)是一种交互式的表,可以动态地改变它的版面布置,以便按照不同方式分析数据,也可以重新安排行号、列标、页字段,比如下面的Excel透视表:
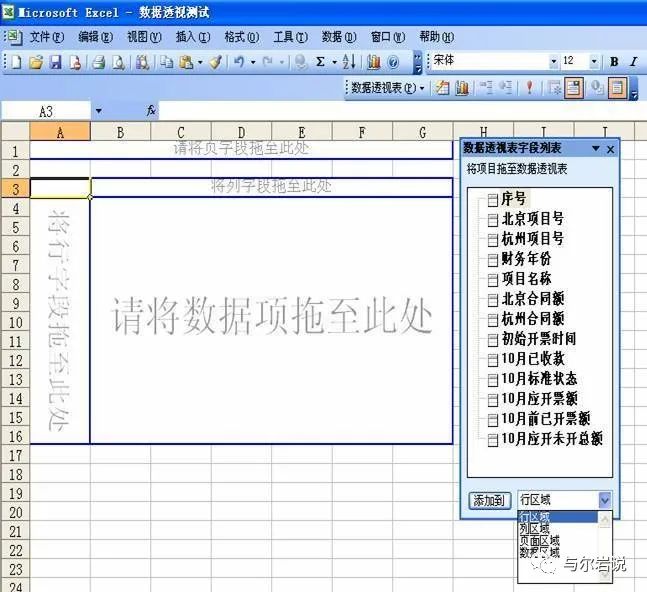
Excel透视表
可以自由选择用来做行号和列标的属性,非常便于我们做不同的分析。Pandas也提供类似的透视表的功能。例如LoanAmount
这个重要的列有缺失值。我们不希望直接使用整体平均值来替换,这样太过笼统,不合理。
这时可以用先根据 Gender
、Married
、Self_Employed
分组后,再按各组的均值来分别替换缺失值。每个组 LoanAmount
的均值可以用如下方法确定:
#Determine pivot table
impute_grps = data.pivot_table(values=["LoanAmount"], index=["Gender","Married","Self_Employed"], aggfunc=np.mean)
print(impute_grps)
LoanAmount
Gender Married Self_Employed
Female No No 114.691176
Yes 125.800000
Yes No 134.222222
Yes 282.250000
Male No No 129.936937
Yes 180.588235
Yes No 153.882736
Yes 169.395833
关键字values
用于指定要使用集成函数(字段aggfunc
)计算的列,选填,不进行指定时默认对所有index以外符合集成函数处理类型的列进行处理,比如这里使用mean
作集成函数,则合适的类型就是数值类型。index
是行标,可以是多重索引,处理时会按序分组。
想了解更多请阅读 Pandas Reference (Pivot Table)
5. 多重索引
不同于DataFrame对象,可以看到上一步得到的Pivot Table每个索引都是由三个值组合而成的,这就叫做多重索引。
从上一步中我们得到了每个分组的平均值,接下来我们就可以用来替换LoanAmount
字段的缺失值了:
#只在带有缺失值的行中迭代:
for i,row in data.loc[data['LoanAmount'].isnull(),:].iterrows():
ind = tuple([row['Gender'],row['Married'],row['Self_Employed']])
data.loc[i,'LoanAmount'] = impute_grps.loc[ind].values[0]
特别注意对Pivot Table使用loc定位的方式,Pivot Table的索引是一个tuple。从上面的代码可以看到,先把DataFrame中所有LoanAmount
字段缺失的数据记录取出,并且使用iterrows函数转为一个按行迭代的对象,每次迭代返回一个索引(DataFrame里的索引)以及对应行的内容。然后从行的内容中把 Gender
、Married
、Self_Employed
三个字段的值提取出放入一个tuple里,这个tuple就可以用作前面定义的Pivot Table的索引了。
接下来的赋值对DataFrame使用loc定位根据索引定位,并且只抽取LoanAmount
字段,把它赋值为在Pivot Table中对应分组的平均值。最后检查一下,这样我们又处理好一个列的缺失值了。
#再次检查缺失值以确认:
print(data.apply(num_missing, axis=0))
Gender 0
Married 0
Dependents 15
Education 0
Self_Employed 0
ApplicantIncome 0
CoapplicantIncome 0
LoanAmount 0
Loan_Amount_Term 14
Credit_History 50
Property_Area 0
Loan_Status 0
dtype: int64
6. 二维表
二维表这个东西可以帮助我们快速验证一些基本假设,从而获取对数据表格的初始印象。例如,本例中Credit_History
字段被认为对会否获得贷款有显著影响。可以用下面这个二维表进行验证:
pd.crosstab(data["Credit_History"],data["Loan_Status"],margins=True)
crosstab
的第一个参数是index,用于行的分组;第二个参数是columns,用于列的分组。代码中还用到了一个margins
参数,这个参数默认为False,启用后得到的二维表会包含汇总数据。
然而,相比起绝对数值,百分比更有助于快速了解数据。我们可以用apply函数达到目的:
def percConvert(ser):
return ser/float(ser[-1])
pd.crosstab(data["Credit_History"],data["Loan_Status"],margins=True).apply(percConvert, axis=1)
从这个二维表中我们可以看到有信用记录 (Credit_History
字段为1.0) 的人获得贷款的可能性更高。接近80%有信用记录的人都 获得了贷款,而没有信用记录的人只有大约8% 获得了贷款。令人惊讶的是,如果我们直接利用信用记录进行训练集的预测,在614条记录中我们能准确预测出460条记录 (不会获得贷款+会获得贷款:82+378) ,占总数足足75%。不过呢~在训练集上效果好并不代表在测试集上效果也一样好。有时即使提高0.001%的准确度也是相当困难的,这就是我们为什么需要建立模型进行预测的原因了。
想了解更多请阅读 Pandas Reference (crosstab)
7. 数据框合并
就像数据库有多个表的连接操作一样,当数据来源不同时,会产生把不同表格合并的需求。这里假设不同的房产类型有不同的房屋均价数据,定义一个新的表格,如下:
prop_rates = pd.DataFrame([1000, 5000, 12000], index=['Rural','Semiurban','Urban'],columns=['rates'])
prop_rates
农村房产均价只用1000,城郊这要5000,城镇内房产比较贵,均价为12000。我们获取到这个数据之后,希望把它连接到原始表格Loan_Prediction_Train.csv中以便观察房屋均价对预测的影响。在原始表格中有一列Property_Area就是表明贷款人居住的区域的,可以通过这一列进行表格连接:
data_merged = data.merge(right=prop_rates, how='inner',left_on='Property_Area',right_index=True, sort=False)
data_merged.pivot_table(values='Credit_History',index=['Property_Area','rates'], aggfunc=len)
Property_Area rates
Rural 1000 179.0
Semiurban 5000 233.0
Urban 12000 202.0
Name: Credit_History, dtype: float64
使用merge函数进行连接,解析一下各个参数:
参数right即连接操作右端表格;
参数how指示连接方式,默认是inner,即内连接。可选left、right、outer、inner;
left: use only keys from left frame (SQL: left outer join)
right: use only keys from right frame (SQL: right outer join)
outer: use union of keys from both frames (SQL: full outer join)
inner: use intersection of keys from both frames (SQL: inner join)
参数left_on用于指定连接的key的列名,即使key在两个表格中的列名不同,也可以通过left_on和right_on参数分别指定。如果一样的话,使用on参数就可以了。可以是一个标签(单列),也可以是一个列表(多列);
right_index默认为False,设置为True时会把连接操作右端表格的索引作为连接的key。同理还有left_index;
sort参数默认为False,指示是否需要按key排序。
所以上面的代码是把data表格和prop_rates表格连接起来。连接时,data表格用于连接的key是Property_Area
,而prop_rates表格用于连接的key是索引,它们的值域是相同的。
连接之后使用了第四小节透视表的方法检验新表格中Property_Area
字段和rates
字段的关系。后面跟着的数字表示出现的次数。
想了解更多请阅读 Pandas Reference (merge)
8. 给数据排序
Pandas可以轻松地基于多列进行排序,方法如下:
data_sorted = data.sort_values(['ApplicantIncome','CoapplicantIncome'], ascending=False)
data_sorted[['ApplicantIncome','CoapplicantIncome']].head(10)
Notice:Pandas 的sort
函数现在已经不推荐使用,使用 sort_values
函数代替。
这里传入了ApplicantIncome
和CoapplicantIncome
两个字段用于排序,Pandas会先按序进行。先根据ApplicantIncome
进行排序,对于ApplicantIncome
相同的记录再根据CoapplicantIncome
进行排序。 ascending参数设为False,表示降序排列。不妨再看个简单的例子:
from pandas import DataFrame
df_temp = DataFrame({'a':[1,2,3,4,3,2,1],'b':[0,1,1,0,1,0,1]})
df_sorted = df_temp.sort_values(['a','b'],ascending=False)
df_sorted
这里只有a和b两列,可以清晰地看到Pandas的多列排序是先按a列进行排序,a列的值相同则会再按b列的值排序。
想了解更多请阅读 Pandas Reference (sort_values)
9. 绘图(箱型图&直方图)
Pandas除了表格操作之外,还可以直接绘制箱型图和直方图且只需一行代码。这样就不必单独调用matplotlib了。
%matplotlib inline
# coding:utf-8
data.boxplot(column="ApplicantIncome",by="Loan_Status")
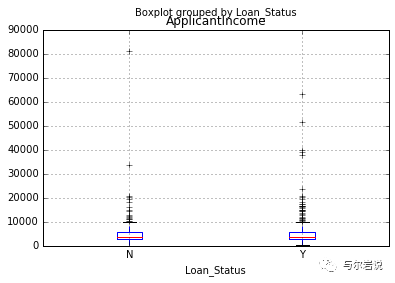
箱型图1
箱型图
因为之前没怎么接触过箱型图,所以这里单独开一节简单归纳一下。
箱形图(英文:Box-plot),又称为盒须图、盒式图、盒状图或箱线图,是一种用作显示一组数据分散情况资料的统计图。因型状如箱子而得名。详细解析看维基百科。
因为上面那幅图不太容易看,用个简单点的例子来说,还是上一小节那个只有a列和b列的表格。按b列对a列进行分组:
df_temp.boxplot(column="a",by="b")
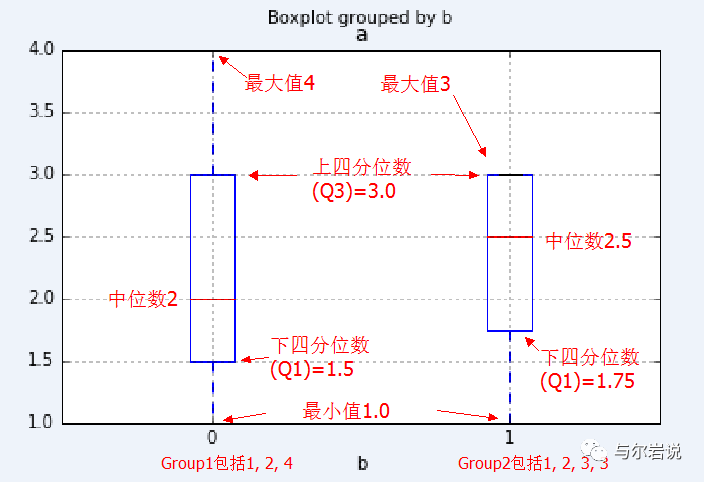
箱型图2
定义b列值为0的分组为Group1,b列值为1的分组为Group2。Group1分组有4,2,1三个值,毫无疑问最大值4,最小值1,在箱型图中这两个值对应箱子发出的虚线顶端的两条实线。Group2分组有3,3,2,1四个值,由于最大值3和上四分位数3(箱子顶部)相同,所以重合了。
Group1中位数是2,而Group2的中位数则是中间两个数2和3的平均数,也即2.5。在箱型图中由箱子中间的有色线段表示。
四分位数
四分位数是统计学的一个概念。把所有数值由小到大排列好,然后分成四等份,处于三个分割点位置的数值就是四分位数,其中:
第一四分位数 (Q1),又称“较小四分位数”或“下四分位数”,等于该样本中所有数值由小到大排列后,在四分之一位置的数。
第二四分位数 (Q2),又称“中位数”,等于该样本中所有数值由小到大排列后,在二分之一位置的数。
第三四分位数 (Q3),又称“较大四分位数”或“上四分位数”,等于该样本中所有数值由小到大排列后,在四分之三位置的数。
Notice:Q3与Q1的差距又称四分位距(InterQuartile Range, IQR)。
计算四分位数时首先计算位置,假设有n个数字,则:
如果n-1恰好是4的倍数,那么数列中对应位置的就是各个四分位数了。但是,如果n-1不是4的倍数呢?
这时位置会是一个带小数部分的数值,四分位数以距离该值最近的两个位置的加权平均值求出。其中,距离较近的数,权值为小数部分;而距离较远的数,权值为(1-小数部分)。
再看例子中的Group1,Q1位置为0.5,Q2位置为1,Q3位置为1.5。(注意:位置从下标0开始!),所以:
Q1 = 0.5*1+0.5*2 = 1.5
Q2 = 2
Q3 = 0.5*2+0.5*4 = 3
而Group2中,Q1位置为0.75,Q2位置为1.5,Q3位置为2.25。
Q1 = 0.25*1+0.72*2 = 1.75
Q2 = 0.5*2+0.5*3 = 2.5
Q3 = 0.25*3+0.75*3 = 3
这样是否就清晰多了XD 然而,四分位数的取法还存在分歧,定义不一,我在学习这篇文章时也曾经很迷茫,直到阅读了源码!!
因为Pandas库依赖numpy库,所以它计算四分位数的方式自然也是使用了numpy库的。而numpy中实现计算百分比数的函数为percentile,代码实现如下:
def percentile(N, percent, key=lambda x:x):
"""
Find the percentile of a list of values.
@parameter N - is a list of values. Note N MUST BE already sorted.
@parameter percent - a float value from 0.0 to 1.0.
@parameter key - optional key function to compute value from each element of N.
@return - the percentile of the values
"""
if not N:
return None
k = (len(N)-1) * percent
f = math.floor(k)
c = math.ceil(k)
if f == c:
return key(N[int(k)])
d0 = key(N[int(f)]) * (c-k)
d1 = key(N[int(c)]) * (k-f)
return d0+d1
读一遍源码之后就更加清晰了。最后举个例子:
from numpy import percentile, mean, median
temp = [1,2,4] # 数列包含3个数
print(min(temp))
print(percentile(temp,25))
print(percentile(temp,50))
print(percentile(temp,75))
print(max(temp))
1
1.5
2.0
3.0
4
temp2 = [1,2,3,3
] # 数列包含4个数
print(min(temp2))
print(percentile(temp2,25))
print(percentile(temp2,50))
print(percentile(temp2,75))
print(max(temp2))
1
1.75
2.5
3.0
3
temp3 = [1,2,3,4,5] # 数列包含5个数
print(min(temp2))
print(percentile(temp3,25))
print(percentile(temp3,50))
print(percentile(temp3,75))
print(max(temp3))
1
2.0
3.0
4.0
5
不熟悉的话就再手撸一遍!!!不要怕麻烦!!!这一小节到此Over~
直方图
data.hist(column="ApplicantIncome",by="Loan_Status",bins=30)
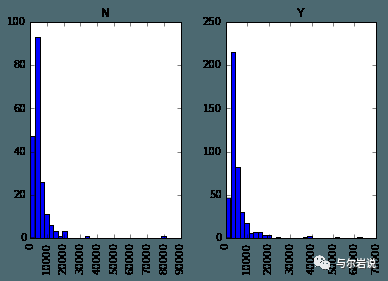
直方图
直方图比箱型图熟悉一些,这里就不详细展开了。结合箱型图和直方图,我们可以看出获得贷款的人和未获得贷款的人没有明显的收入差异,也即收入不是决定性因素。
离群点
特别地,从直方图上我们可以看出这个数据集在收入字段上,比较集中于一个区间,区间外部有些散落的点,这些点我们称为离群点(Outlier)。前面将箱型图时没有细说,现在再回顾一下箱型图:
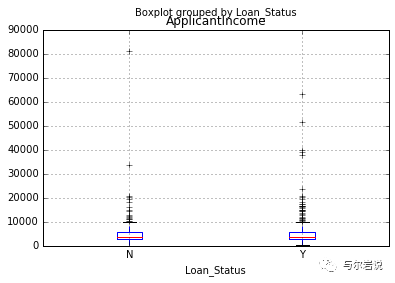
箱型图1
可以看到除了箱子以及最大最小值之外,还有很多横线,这些横线其实就表示离群点。那么可能又会有新的疑问了?怎么会有离群点在最大最小值外面呢?这样岂不是存在比最大值大,比最小值小的情况了吗?
其实之前提到一下,有一个概念叫四分位距(IQR),数值上等于Q3-Q1,记作ΔQ。定义:
最大值区间:Q3+1.5ΔQ
最小值区间:Q1-1.5ΔQ
也就是说最大值必须出现在这两个区间内,区间外的值被视为离群点,并显示在图上。这样做我们可以避免被过分偏离的数据点带偏,更准确地观测到数据的真实状况,或者说普遍状况。
想了解更多请阅读 Pandas Reference (hist) | Pandas Reference (boxplot)
10. 用Cut函数分箱
有时把数值聚集在一起更有意义。例如,如果我们要为交通状况(路上的汽车数量)根据时间(分钟数据)建模。具体的分钟可能不重要,而时段如“上午”“下午”“傍晚”“夜间”“深夜”更有利于预测。如此建模更直观,也能避免过度拟合。
这里我们定义一个简单的、可复用的函数,轻松为任意变量分箱。
#分箱:
def binning(col, cut_points, labels=None):
#Define min and max values:
minval = col.min()
maxval = col.max()
#利用最大值和最小值创建分箱点的列表
break_points = [minval] + cut_points + [maxval]
#如果没有标签,则使用默认标签0 ... (n-1)
if not labels:
labels = range(len(cut_points)+1)
#使用pandas的cut功能分箱
colBin = pd.cut(col,bins=break_points,labels=labels,include_lowest=True)
return colBin
#为年龄分箱:
cut_points = [90,140,190]
labels = ["low","medium","high","very high"]
data["LoanAmount_Bin"] = binning(data["LoanAmount"], cut_points, labels)
print(pd.value_counts(data["LoanAmount_Bin"], sort=False))
low 104
medium 273
high 146
very high 91
dtype: int64
解析以下这段代码,首先定义了一个cut_points列表,里面的三个点用于把LoanAmount
字段分割成四个段,对应地,定义了labels列表,四个箱子(段)按贷款金额分别为低、中、高、非常高。然后把用于分箱的LoanAmount
字段,cut_points,labels传入定义好的binning函数。
binning函数中,首先拿到分箱字段的最小值和最大值,把这两个点加入到break_points列表的一头一尾,这样用于切割的所有端点就准备好了。如果labels没有定义就默认按0~段数-1命名箱子。最后借助pandas提供的cut函数进行分箱。
cut函数原型是cut(x, bins, right=True, labels=None, retbins=False, precision=3, include_lowest=False)
,x是分箱字段,bins是区间端点,right指示区间右端是否闭合,labels不解释..retbins表示是否需要返回bins,precision是label存储和显示的精度,include_lowest指示第一个区间的左端是否闭合。
在把返回的列加入到data后,使用value_counts函数,统计了该列的各个离散值出现的次数,并且指定不需要对结果进行排序。
想了解更多请阅读 Pandas Reference (cut)
11.为分类变量编码
有时,我们会面对要改动分类变量的情况。原因可能是:
有些算法(如logistic回归)要求所有输入项目是数字形式。所以分类变量常被编码为0, 1….(n-1)
有时同一个分类变量可能会有两种表现方式。如,温度可能被标记为“High”, “Medium”, “Low”,“H”, “low”。这里 “High” 和 “H”都代表同一类别。同理, “Low” 和“low”也是同一类别。但Python会把它们当作不同的类别。
一些类别的频数非常低,把它们归为一类是个好主意。
这里我们定义了一个函数,以字典的方式输入数值,用‘replace’函数进行编码
#使用Pandas replace函数定义新函数:
def coding(col, codeDict):
colCoded = pd.Series(col, copy=True)
for key, value in codeDict.items():
colCoded.replace(key, value, inplace=True)
return colCoded
#把贷款状态LoanStatus编码为Y=1, N=0:
print('Before Coding:')
print(pd.value_counts(data["Loan_Status"]))
data["Loan_Status_Coded"] = coding(data["Loan_Status"], {'N':0,'Y':1})
print('\nAfter Coding:')
print(pd.value_counts(data["Loan_Status_Coded"]))
Before Coding:
Y 422
N 192
Name: Loan_Status, dtype: int64
After Coding:
1 422
0 192
Name: Loan_Status_Coded, dtype: int64
在coding函数中第二个参数是一个dict,定义了需要编码的字段中每个值对应的编码。
实现上首先把传入的列转换为Series对象,copy参数表示是否要进行复制,关于copy可以看我另一篇笔记:深拷贝与浅拷贝的区别。
copy得到的Series对象不会影响到原本DataFrame传入的列,所以可以放心修改,这里replace函数中的inplace参数表示是否直接在调用对象上作出更改,我们选择是,那么colCoded对像在调用replace后就会被修改为编码形式了。最后,把编码后的列加入到data中,比较编码前后的效果。
想了解更多请阅读 Pandas Reference (replace)
12. 在一个数据框的各行循环迭代
有时我们会需要用一个for循环来处理每行。比方说下面两种情况:
带数字的分类变量被当做数值。
带文字的数值变量被当做分类变量。
先看看data表格的数据类型:
#检查当前数据类型:
data.dtypes
结果:
Gender object
Married object
Dependents object
Education object
Self_Employed object
ApplicantIncome int64
CoapplicantIncome float64
LoanAmount float64
Loan_Amount_Term float64
Credit_History float64
Property_Area object
Loan_Status object
LoanAmount_Bin category
Loan_Status_Coded int64
dtype: object
可以看到Credit_History这一列被当作浮点数,而实际上我们原意是分类变量。所以通常来说手动定义变量类型是个好主意。那这种情况下该怎么办呢?这时我们需要逐行迭代了。
首先创建一个包含变量名和类型的csv文件,读取该文件:
#载入文件:
colTypes = pd.read_csv(r'F:\Datahack_Loan_Prediction\datatypes.csv')
print(colTypes)
feature type
0 Loan_ID categorical
1 Gender categorical
2 Married categorical
3 Dependents categorical
4 Education categorical
5 Self_Employed categorical
6 ApplicantIncome continuous
7 CoapplicantIncome continuous
8 LoanAmount continuous
9 Loan_Amount_Term continuous
10 Credit_History categorical
11 Property_Area categorical
12 Loan_Status categorical
载入这个文件之后,我们能对它的逐行迭代,然后使用astype函数来设置表格的类型。这里把离散值字段都设置为categorical类型(对应np.object),连续值字段都设置为continuous类型(对应np.float)。
#迭代每行,指派变量类型。
#注,astype函数用于指定变量类型。
for i, row in colTypes.iterrows(): #i: dataframe索引; row: 连续的每行
if row['type']=="categorical" and row['feature']!="Loan_ID":
data[row['feature']]=data[row['feature']].astype(np.object)
elif row['type']=="continuous":
data[row['feature']]=data[row['feature']].astype(np.float)
print(data.dtypes)
Gender object
Married object
Dependents object
Education object
Self_Employed object
ApplicantIncome float64
CoapplicantIncome float64
LoanAmount float64
Loan_Amount_Term float64
Credit_History object
Property_Area object
Loan_Status object
dtype: object
可以看到现在信用记录(Credit_History
)这一列的类型已经变成了‘object’ ,这在Pandas中代表分类变量。
特别地,无论是中文教程还是原版英文教程这个地方都出错了.. 中文教材代码中判断条件是错的,英文教程中没有考虑到Loan_ID
这个字段,由于它被设定为表格的索引,所以它的类型是不被考虑的。
想了解更多请阅读 Pandas Reference (iterrows)
三、 总结
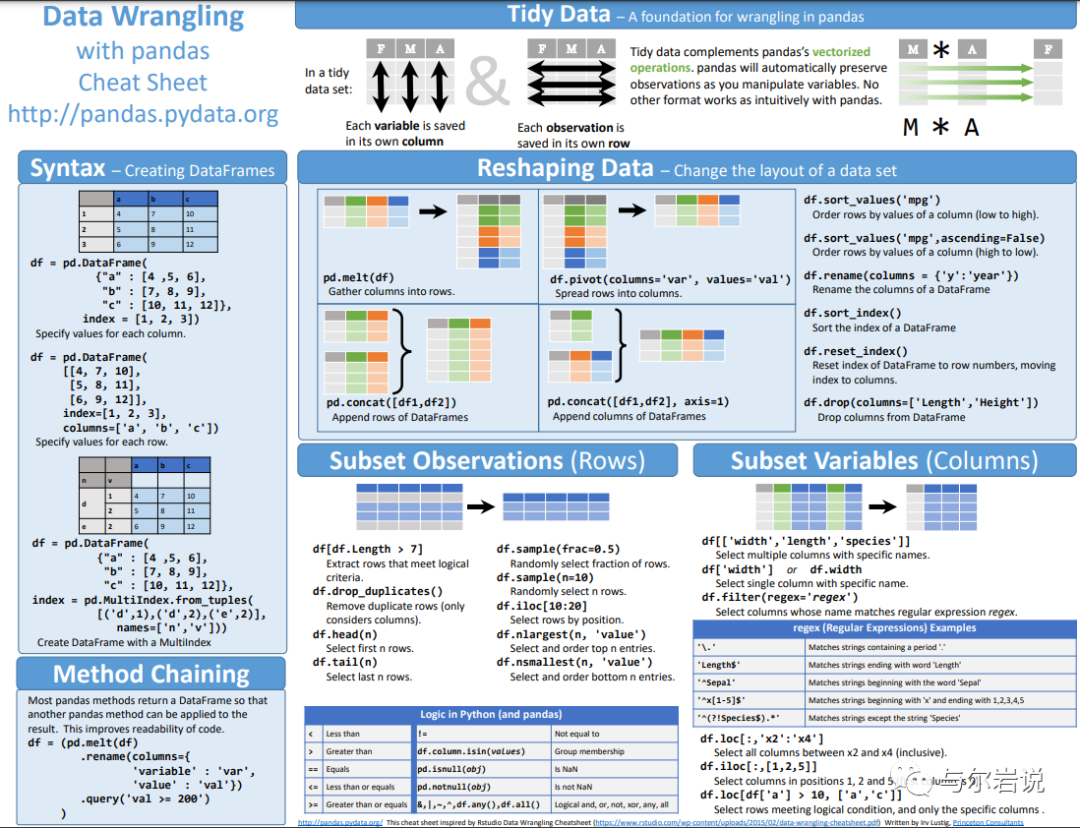
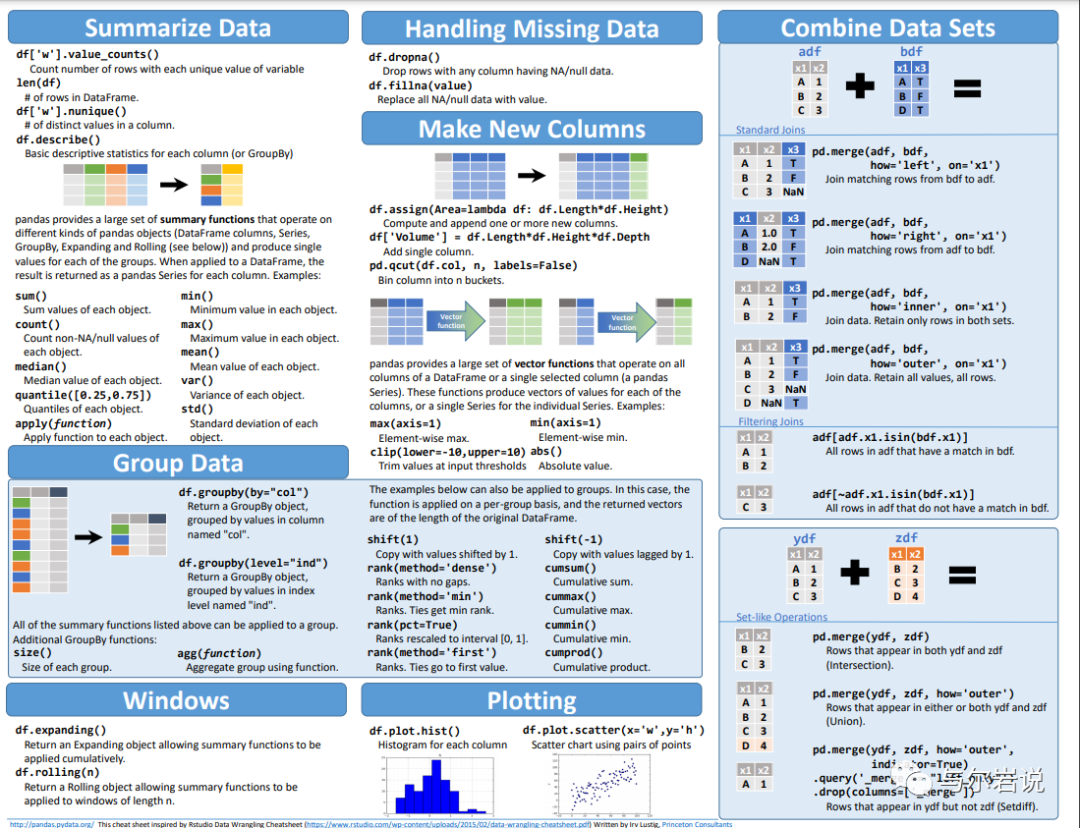